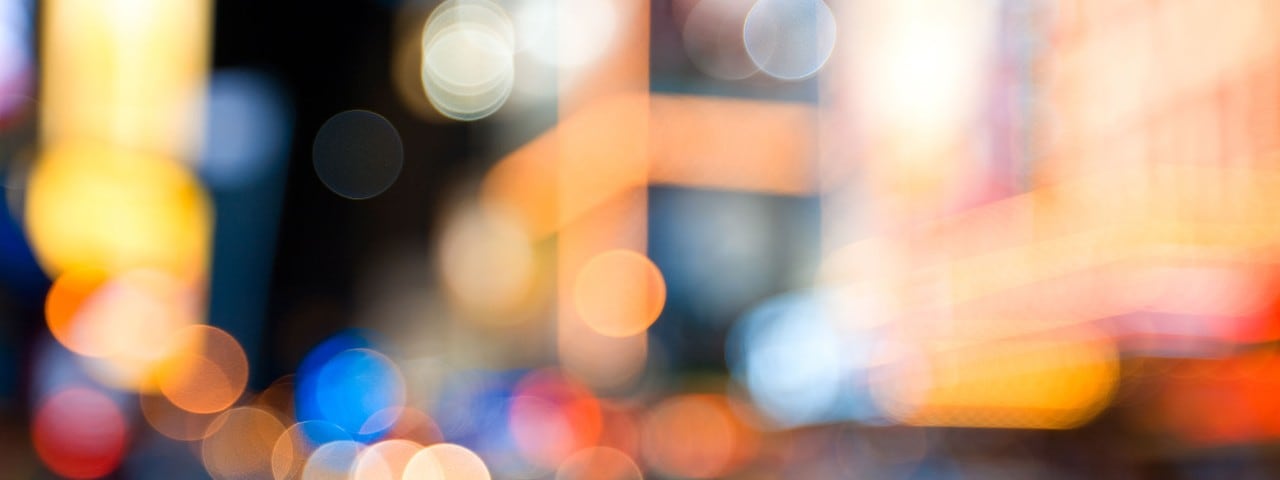
Homelessness data holds insights to a hidden problem
By: Kara Roberts, SAS Corporate & Executive Communications
Mary* was a middle school teacher in Cary, NC, with more than 20 years of classroom experience. After tragically experiencing domestic violence in her home, Mary entered a bitter divorce, which left her and her two sons homeless.
Many people stereotype the homeless as jobless, addicts or criminals. Mary was none of those things. And the truth is, many families stumble upon bad fate including sickness, divorce, job loss or domestic violence, and find themselves without a home.
Overwhelmed and scared, Mary turned to one of her school counselors to ask for help. The counselor suggested she contact The Carying Place to find support and guidance to help regain her confidence and get her family into the home they deserved.
The Carying Place (TCP) is an organization that has supported working homeless families like Mary’s for 27 years. More than 400 families have completed TCP’s 16-week program, which provides short-term, transitional housing and life skills training for homeless families. By empowering and equipping families for independent living, TCP helps break the cycle of poverty and gives families a chance to reach self-sufficiency.
Uncovering the struggles of the “hidden homeless” who are right in front of us helps us understand the full picture of homelessness in our community so organizations like TCP can better serve these individuals. Thanks to a partnership with SAS, TCP has a new way to measure indicators of participant success to provide these families the help that they deserve.
Learn more about how SAS is using data for good
From preventing life-threatening illnesses to protecting endangered species to rebuilding after natural disasters, organizations across the globe are harnessing data to make a difference.
Celebrating Mary’s success
After being accepted into the program, Mary and her family were placed in an all-expenses paid housing unit by TCP. A volunteer support team provided Mary with weekly guidance in managing personal finances, setting attainable goals, seeking permanent affordable housing and maintaining employment. After setting clear goals of where she wanted to be, Mary worked diligently for four months, guided and mentored by her volunteer team and past program graduates, to save money, relearn key skills and become self-sufficient.
Not only did Mary successfully complete the program and move to a place of her own at its conclusion, but today she acts as a graduate mentor, speaking at workshops and helping inspire the “hidden homeless” who have gone through experiences similar to hers.
Mary recently bought a house of her own thanks in part to the money she saved and the resources that she received throughout the program. She now uses her experience to help others, hosting presentations to current TCP families about the process she followed to achieve this milestone.
“That’s the ability that The Carying Place lends to families. We don’t do the work. We give them the structure to do the work. And they do it. This can be the result,” said Leslie Covington, Executive Director of TCP. “The ability for her to be that successful inspires other families to strive for that.”
Answering questions with data
To better serve families like Mary’s, TCP knew it needed a stronger strategy to collect and analyze its 27 years’ worth of data. Like many nonprofit organizations, TCP wanted to have data to validate the program’s success, but it didn’t have the systems or processes in place for detailed data analysis nor the budget to hire an external group to perform the work.
TCP had questions and hoped that answers could be hidden within its data:
- What are the common characteristics of those who are graduating from the program?
- Would the program be more successful if it were a few months longer?
- What other partners should we introduce to assist families?
With all these questions, piles of hand-written data and inconsistent Excel spreadsheets, Carolyn Hendricks, a Senior IT Manager at SAS and a member of the TCP Board of Directors, suggested that TCP let SAS lend a hand.
"Our priority is to help families through the hardest seasons of life, and much of that work is supporting the homeless who are hidden in plain sight,” Covington said. “That’s what we do best. SAS was able to take the data right in front of us and uncover hidden insights we couldn’t see before. That’s what they do best. It was honestly the perfect combination.”
Our priority is to help families through the hardest seasons of life, and much of that work is supporting the homeless who are hidden in plain sight. That’s what we do best. SAS was able to take the data right in front of us and uncover hidden insights we couldn’t see before. That’s what they do best. It was honestly the perfect combination. Leslie Covington Executive Director The Carying Place
For TCP to see the full story of its data, it first had to understand data fundamentals. Covington describes it as a four-step process:
- Standardize the data
The first step in the data analysis process and the first major lesson for TCP was standardizing the data. With various volunteers entering information and no clear standard, TCP had data sets that were mismatched and hard to analyze.
A team of SAS analytic volunteers sorted through TCP’s data, recoded and normalized it to draw better conclusions about the program. The main data sources they cleaned and used for analysis were paper forms and Excel spreadsheets of information about each family including demographics, insurance, housing, veteran status, disability status and budget files.
The team cleansed the data and standardized various fields, such as education level, support system and referral source, to allow accurate conclusions to be drawn. Additionally, they joined data sources together with one row per family to allow the TCP team to clearly understand the best indicators of success.
- Choose a model
The team then needed to choose the best model to describe the data, which is an essential step in data fundamentals. Effectively finding answers to an organization’s questions is dependent on finding the best-suited model for the project that also allows for future growth. The team explored which models would be most effective for understanding significant factors of participant success, and after trying various machine learning and advanced analytics options, chose decision trees as the champion model. This model considers all the factors that TCP collects about each family and presents a clear visual representation of how each factor generally impacts success rates.
- Gather results
Overall, TCP’s success was validated – the program is working and is helping lead families on a path to independent living. Specific results from the analysis included several data points that showed correlation between family background and graduation rates, such as the fact that families with higher education levels tend to be most successful in the program.
Other revelations were less obvious. For example, because the housing TCP provides does not allow for pets, the data revealed that families who enter the program with pets do not do as well. This finding allowed TCP to brainstorm ways to remove similar barriers and improve the chances of graduating for families with pets.
“The data made us ask all kinds of questions and made us become more strategic in how we are determining any changes within our program. It made us slow down and say, ‘Let’s record the data on this for a while longer to see if that change is really what we need,’” said Covington.
- Keep collecting and improving
With a better understanding of data fundamentals and the importance of standardized data, TCP is continuing to collect information about families, but this time in a cleaner and more consistent way. This will allow TCP to draw more statistically sound conclusions and make significant, data-driven change within the organization.
In the meantime, TCP is making adjustments based on findings from this project to better serve its community. After validating that the majority of its families are led by single-family mothers, TCP has been able to adjust where it finds program participants by strategically targeting locations where these mothers may be seeking help.
TCP now understands the cyclical nature of analytics – it is a journey, not a destination. In order to continue to grow and improve its services, TCP will continue moving through the analytics life cycle to make better, data-driven decisions.
TCP isn't just helping the homeless as we've defined for ourselves; TCP is also caring for those who we don’t even realize are homeless – from stylists doing our hair to teachers educating our children.
Now, TCP can better support all people with the help of basic analytics – no complex analytical models or artificial intelligence required. With just a newfound understanding of data fundamentals, the help of talented volunteers and a pure passion for helping others, TCP is helping give more “hand ups, not handouts” to families like Mary’s and hundreds of others in our community.
Recommended reading
-
Data-driven health careDiscover the world of data-driven healthcare as we explore some inspiring stories of how healthcare providers are using big data analytics to tackle global health issues.
-
Analytics for prescription drug monitoring: How to better identify opioid abusePrescription drug monitoring programs (PDMPs) are a great start in combating abuse of prescription drugs, but they could be doing much more. Better data and analytics can inform better treatment protocols, provider education and policy decisions – and save lives.
-
From living on the streets to owning a profitable businessZoe Empowers, a ministry for vulnerable children living in life-threatening poverty in Africa and India, finally found a way to measure and reveal its incredible impact. Using SAS, they developed an "empowerment index" that measures improvements in housing, health, education and more for program participants.