Il deep learning nel mondo di oggi
L'impatto del deep learning è piuttosto significativo. E siamo solo all'inizio.
Il deep learning con SAS e Python
DLPy è un pacchetto open source che i data scientist possono scaricare per applicare algoritmi di deep learning SAS a dati di immagini, testo e audio. È stato progettato per risolvere problemi di computer vision, elaborazione del linguaggio naturale, forecasting ed elaborazione del linguaggio parlato.
In che modo SAS utilizza il deep learning
Questa guida confronta diversi modelli di rete neurale e spiega come usarli, passo dopo passo. Ti introdurrà alle tecniche di deep learning, alle sue applicazioni e al modo in cui SAS supporta la creazione di modelli di reti neurali profonde.
Come utilizzare il deep learning per l'embedding delle immagini
I modelli di embedding vengono utilizzati per ridurre la dimensionalità dei dati di input, come le immagini. Quando si utilizza un modello di embedding, le immagini di input vengono convertite in vettori a bassa dimensione, che sono più facilmente utilizzabili per altre attività di computer vision. Il segreto per un embedding di successo è addestrare il modello a convertire immagini simili in vettori simili.
Come funziona il deep learning
Il deep learning cambia il tuo modo di affrontare le sfide risolvibili con gli analytics. Mentre prima si diceva al computer come risolvere un determinato problema, ora lo si addestra a risolvere il problema stesso.
Rappresentazione delle caratteristiche
Il deep learning introduce un cambio di paradigma nella costruzione dei modelli, perché segna il passaggio dall'ingegneria delle caratteristiche alla rappresentazione delle caratteristiche.
Layer del deep learning
Invece di utilizzare le variabili note per prevedere le incognite, il deep learning utilizza i layer dei dati per riconoscerne le caratteristiche latenti.
I risultati del deep learning
Il deep learning è in grado di fornire un sistema predittivo che generalizza bene, si adatta con facilità e migliora di continuo grazie all’immissione di nuovi dati. Non è più necessario adattarsi a un modello. È invece possibile addestrarlo a un compito.
L'approccio tradizionale agli analytics consiste nell'utilizzare i dati a disposizione per progettare funzionalità da cui derivare nuove variabili, quindi selezionare un modello analitico e infine stimare i parametri (o le incognite) di quel modello. Queste tecniche possono dare origine a sistemi predittivi che non generalizzano bene, poiché la completezza e la correttezza dipendono dalla qualità del modello e dalle sue caratteristiche. Ad esempio, se si sviluppa un modello di frode con il feature engineering, si parte da un insieme di variabili da cui probabilmente si ricaverà un modello utilizzando le trasformazioni dei dati. Ci si può ritrovare con un modello che dipende da 30.000 variabili. A quel punto, occorre dare una forma al modello, individuare tra le variabili quelle significative e così via. Se si aggiungono ulteriori dati, occorre ripetere il lavoro daccapo.
Il nuovo approccio basato sul deep learning consiste nel sostituire la formulazione e la specificazione del modello con caratterizzazioni gerarchiche (o strati), che imparano a riconoscere le caratteristiche latenti dei dati dalle regolarità negli strati.
Gli Advanced Analytics SAS
Il deep learning è solo uno degli strumenti a disposizione dei data scientist. Scopri le altre soluzioni di advanced analytics, tra cui forecasting, text analytics e optimization.
Letture consigliate
-
ARTICOLO Cosa sono le allucinazioni dell'AI?Può essere difficile distinguere la realtà dalla finzione generata dall'intelligenza artificiale. Scopri perché i modelli linguistici di grandi dimensioni possono sbagliare e provocare le allucinazioni dell'AI, e impara a utilizzare la GenAI in modo responsabile.
-
ARTICOLO 4 strategies that will change your approach to fraud detectionAs fraudulent activity grows and fighting fraud becomes more costly, financial institutions are turning to anti-fraud technology to build better arsenals for fraud detection. Discover four ways to improve your organization's risk posture.
-
ARTICOLO Artificial Intelligence oltre l’HypeSette triliardi di dispositivi connessi da qui al 2025. La capacità di computing e di analisi sarà distribuita sulla superficie di questi sensori. I motori di analisi costruiti su reti neurali e algoritmi di machine learning saranno alla base di questo nuovo ecosistema.
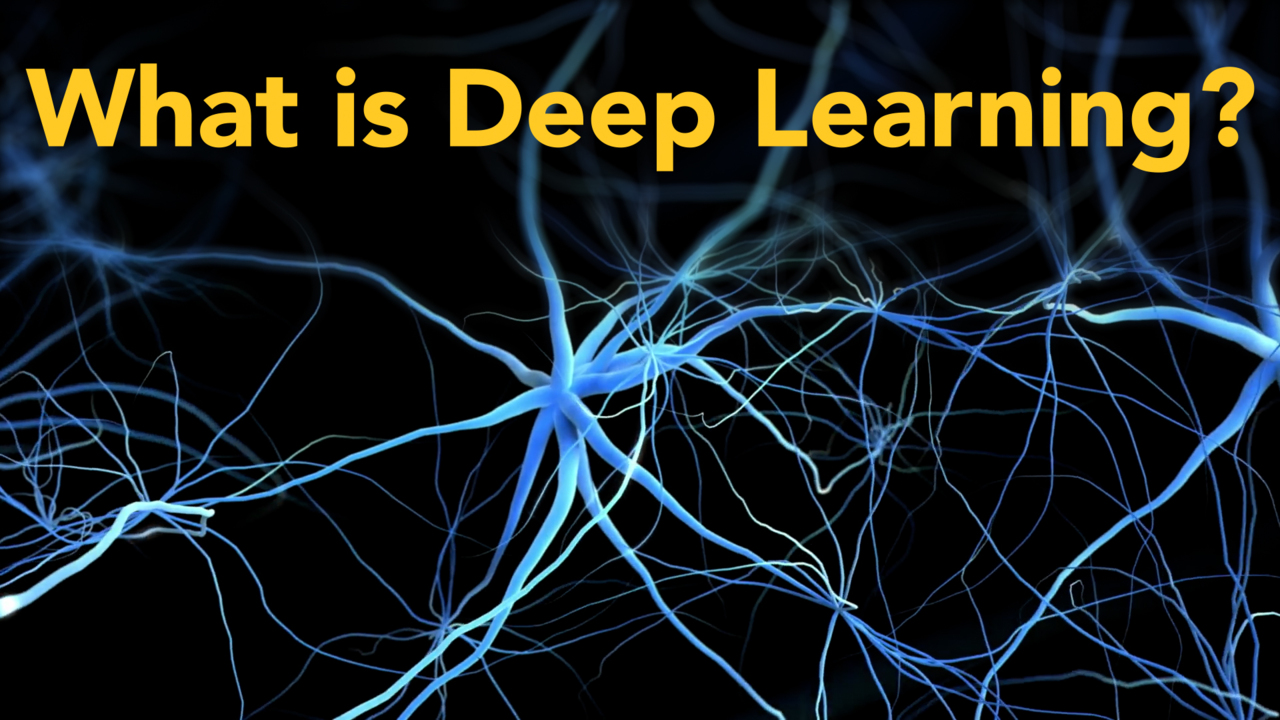