From lab to life
Five tips for operationalizing analytics
By David Wallace, Global Financial Services Marketing Manager, SAS
Thanks to new technologies, building good models is faster and easier than it used to be. What used to take six months to a year can now – with the right information – take just a day, a week or a month.
But getting models deployed is a different story. How long does it take? In the financial services industry, it could take from six months to five years. Even worse, many organizations have models just sitting on a shelf in a lab, not being used at all. How do you take models out of the lab – where they’re in a nice, tidy environment – and introduce them to the real world?
Aren Arakelyan, Assistant Vice President of Credit Risk at M&T Bank, and John Lodmell, Director of Credit and Data Analytics at Advance America, recently shared their best practices in a panel presentation to financial services executives.
Here are five tips they offered:
- Make sure everyone has the same goal in mind.
Consider, for example, Advance America, a payday lender. The company had multiple systems and data scattered across its headquarters and 3,000 stores when it hired Lodmell. His goal was to modify models used at headquarters to change behaviors at the stores and encourage the desired business results.
There are always competing interests and people who are against changing the way they work. An executive sponsor, and ideally your company culture, can help you manage that.
Aren Arakelyan
AVP of Credit Risk
M&T Bank
“We needed to get the stores to be smarter – and be consistent – so we could learn from what’s going on, and pick up on trends, pick up on risk pieces,” Lodmell said. To do it, he had to bring the analytics team and executives alike on board with his plans.
- Get solid executive support.
“There are always competing interests and people who are against changing the way they work,” Arakelyan said. “An executive sponsor, and ideally your company culture, can help you manage that.”
What’s the key to getting that buy-in? According to Lodmell you should “look for ways to get some quick wins.” He’s found that those wins show leaders that the change is working.
“But your strategy needs to be very specific – such as optimizing a certain decision – and it needs to be clear how you’re doing it and what the expected outcome is,” Lodmell said. “Still, you shouldn’t expect quick change. People won’t show up at work on Monday morning and make analytics a key strategic component from that moment on. Change takes time.”
- Prove that the model can be a better decision maker than the person.
People who are accustomed to having the power to make decisions don’t like giving up control, and it takes time to demonstrate that the model can recognize patterns better than people. Consider compliance for example. In his heavily regulated market, Lodmell chose not to allow overrides because there were too many questions about how to make sure they were done consistently and fairly.
According to Lodmell, models take out the human subjectivity that makes it hard for regulators to know if you’re being fair. But if you use a model with no human overrides, as Advance America does, there’s no question about why a loan was, or was not, approved.
The sales organization was resistant. But after Lodmell proved that the models performed better than the overrides, the salespeople no longer complained. They knew the model worked.
- Educate, and be educated by, the stakeholders.
You need to explain to stakeholders that models are based on what has actually happened. And that you developed a model that can best predict what will happen next.
A model used at Advance America, for example, considers the distance between the customer’s residence and the store. Why? Because fraud is likely to be involved if someone drives a long distance to get a payday loan. Clearly, this is just one attribute, and it can’t account for all fraud schemes – but it was selected because it’s a reliable fraud indicator.
During the final stage of model attribute selection at M&T Bank, business experts assess the chosen attributes. As it turns out, their opinions are vital. Don’t hesitate to supplement your analytic processes with the valuable insights of longtime experts. “They’ve been doing things successfully for years,” Arakelyan said. “It also helps to melt the ice, so to speak. And by including them in the process, they’re more likely to understand the valuable role analytics can play in their jobs.”
- Connect the right and left sides of the business brains.
Statisticians often view their efforts as academic endeavors, and they don’t necessarily want to understand all of the business processes involved. Business people are often content to leave the statisticians in a back room and call them out only when they need a magic bullet. Closing that gap between both sets of experts is key to successful model deployment.
One way to do it is by adding business analysts or data scientists to the team – people who are knowledgeable about the data as well as how the business works. They can serve as translators between the statistician and the business executive (who has a business goal in mind, such as reducing risk). They can explain to the statistician what the executive means, describe what needs to change and help extract the right information to build relevant models.
In recent years, companies like SAS have created capabilities and that let you build models with user-friendly interfaces. The ease of turning out effective models quickly makes it easier to show how your models have improved ROI. With proven business results, an organization will be more inclined to use those models on a wider basis.
What are the implications of moving modeling into the mainstream of your organization? When you make model development and implementation an important element in strategic and tactical decision-making processes of your organization, those decisions can be made with more confidence. You can then focus on activities than further boost your bottom line – pricing, profitability and, of course, next-generation analytics.
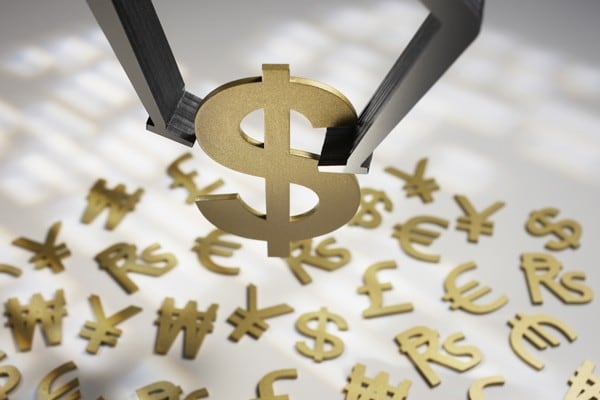
Read More
- Return here for insightful articles, videos and more about the latest trends and topics in analytics.