Big data integration: Go beyond 'just add data'
by Matthew Magne, SAS Global Product Marketing Manager for Data Management
You have probably been in my seat, listening to a keynote presenter at a conference talking about how the “next big thing” was going to “revolutionize the way you do business.” The technology would take all the data that you have, make sense of it, optimize those pesky business processes, and spit out accurate reports and dashboards.
All you had to do was “just add data.” It’s as simple as that.
The trouble is, after a couple of decades, all these technologies labeled as the next big thing have started to blur. Was it a data warehouse? A CRM system? An ERP system? Maybe MDM? Now, is it Hadoop or a data lake?
Not surprisingly, you could swap out the technology and the presentations would sound the same. And each time, I would watch organizations big and small scramble to catch the next wave. The results were often a bit murky, though. Organizations invest time and resources into the next big thing but rarely see the intended results.
That leads me to wonder: Why does the technology world (and its consumers) continue to chase the next revolution? And perhaps more importantly, what’s keeping us from the nirvana we assume is right around the corner?
The answer is almost always that we underestimate the “just add data” phase. That’s the hard part. To be more precise, that’s the incredibly difficult part that involves internal politics, organizational change and other things that aren’t related directly to the application – but can cause it to fail and fail fast.
A new white paper – Data Integration Déjà Vu: Big Data Reinvigorates DI – explores the role of big data integration. It applies the tried-and-true processes from data integration (that are almost as old as computers themselves) to more modern, big data environments. It examines how the more things change, the more they stay the same. Reliable, accurate and consistent data is a requirement for everything that will come next – analytics, dashboards and business process optimization.
The following excerpt details how data integration is adapting to today’s always-on, complex and massive data environments.
Data integration adapts to change
Data integration started way back when organizations realized they needed more than one system or data source to manage the business. With data integration, organizations could combine multiple data sources together. And data warehouses frequently used data integration techniques to consolidate operational system data and to support reporting or analytical needs.
But things kept getting more complex. When it became clear that the huge number of applications, systems and data warehouses had created a smorgasbord of data that was challenging to maintain, enterprise architects started to create smarter frameworks to integrate data. They created canonical models, batch-oriented ETL/ELT (extract-transform-load, extract-load-transform), service oriented architecture, the enterprise service bus, message queues, real-time web services, semantic integration using ontologies, master data management and more.
After all this time and with all these mature technologies in place, why would we still need new data integration paradigms? Why do organizations keep investing in this software?
It comes down to these three trends:
- Increasing numbers of indigenous and exogenous data sources that organizations use for competitive advantage, including social media, unstructured text and sensor data from smart meters and other devices.
- Unprecedented rate of growth in data volumes.
- Emerging technologies like Hadoop that expand beyond the reach of traditional data management software.
These trends have put tremendous pressure on existing infrastructures, pushing them to do things they were never intended to do. Bound by inflexible techniques in the face of big data, many organizations find it nearly impossible to make full use of all their data. On top of that, they need to keep an eye on the emergence of logical data warehousing, the necessary cohabitation of integration patterns, and the new capabilities required to support those requirements – such as Hadoop, NoSQL, in-memory computing and data virtualization.

Matthew is a connector, avid Catan player and the Global Product Marketing Manager for SAS Data Management, focusing on big data, master data management, data quality, data integration and data governance. Previously, Matthew was an Information Management Solutions Architect at SAS, worked as a Certified Data Management Consulting IT Professional at IBM, and is a recovering software engineer and entrepreneur.
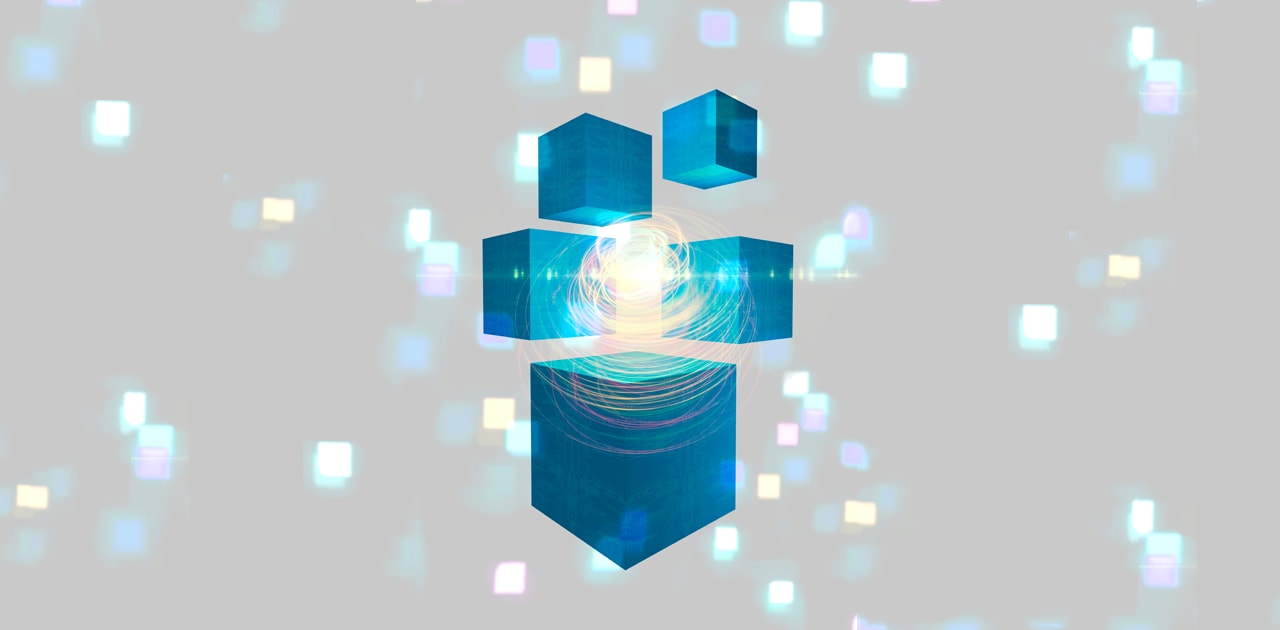
Read More
Learn how data integration is adapting to big data environments.
Related Articles
Get More Insights
Want more Insights from SAS? Subscribe to our Insights newsletter. Or check back often to get more insights on the topics you care about, including analytics, big data, data management, marketing, and risk & fraud.