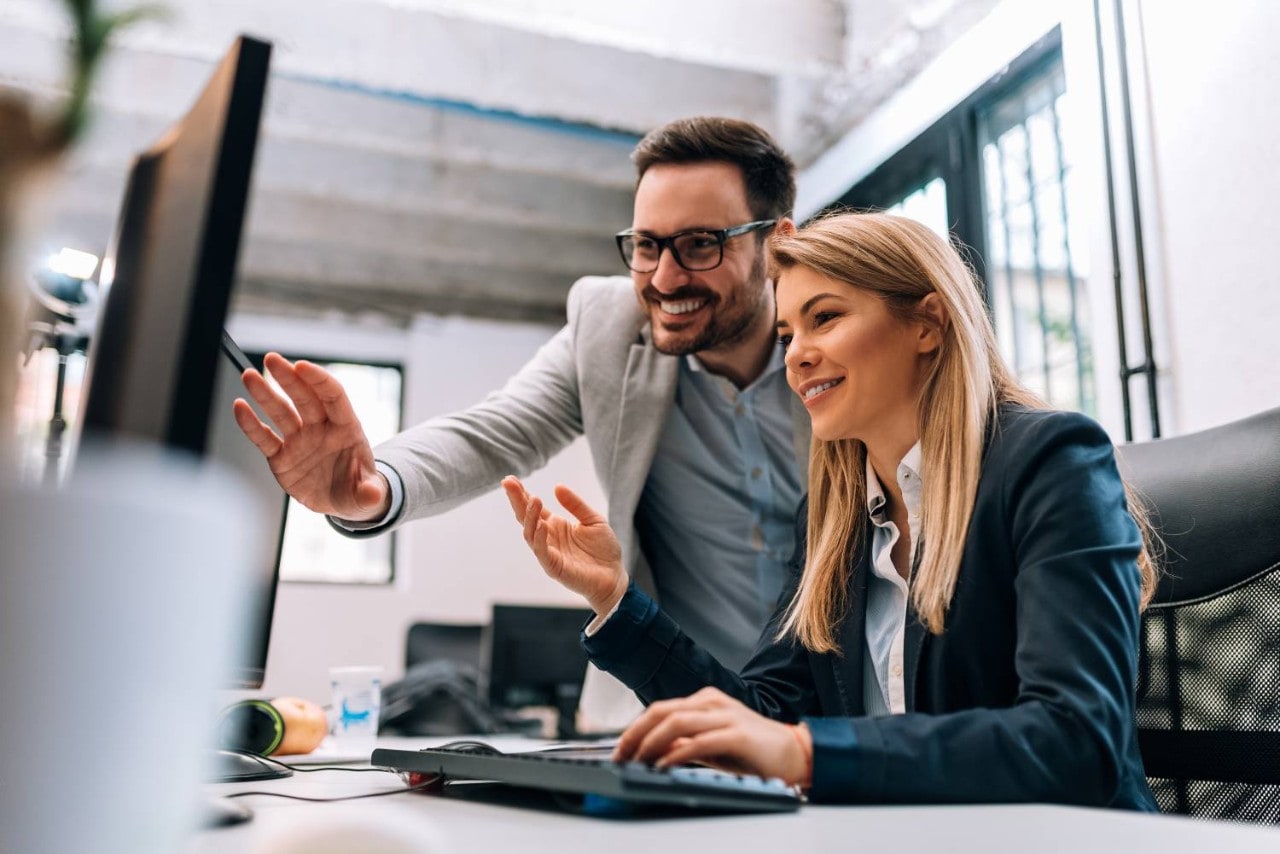
데이터 사이언티스트, MLOps 엔지니어, 비즈니스 분석가를 서로 연결하고, 신속하게 모델을 배포하고, 오픈소스와 통합합니다.
모델 거버넌스와 투명성 보장
모든 유형의 모델과 분석 자산을 검색할 수 있는 중앙집중식 리포지토리가 분석 프로세스에 대한 완전한 가시성을 제공하고, 추적성과 거버넌스를 보장합니다. 이 솔루션은 버전 제어를 통해 모델 관리를 단순화합니다. 또한 모델 관리 프로세스의 각 단계에서 프로젝트 기록을 추적하고, 각 모델의 최신성과 정의 및 가치에 대한 종합적 뷰를 얻을 수 있습니다. 개방형 REST API를 사용하여 모델과 모델 스코어 아티팩트에 액세스하므로 IT 작업이 간소화됩니다.
간편한 방식의 모델 검증으로 고품질 예측
SAS의 오픈소스 패키지인 sasctl을 사용하면 Python 기반 모델에 대한 실행 가능한 스코어링 코드를 자동으로 생성할 수 있습니다. 사용이 간편한 노코드 인터페이스로 모델을 쉽게 테스트할 수 있으며, 프로덕션으로 푸시하기 전에 모델 스코어링 논리를 검증할 수 있습니다.
한 번 구축하면 재코딩 없이 어디든 배포
조직의 이노베이션 랩에서 만든 분석 모델을 원하는 프로덕션 환경으로 효율적으로 이동시킬 수 있습니다. SAS Model Manager는 그 어떤 요구사항도 두루 지원합니다. 예를 들어 데이터베이스에 모델을 배포하는 것은 물론, 일괄 처리 방식의 데이터 스코어링, 실시간 REST API 스코어링 엔드포인트 호스팅, 그리고 Docker, Azure, GCP, AWS의 레지스트리에서 호스팅되는 컨테이너로의 모델 푸시, Azure Machine Learning에 대한 직접 배포 등이 가능합니다.
모델 성능을 자동으로 모니터링하여 의도한 성능 유지
SAS Model Manager는 모델 생성에 사용된 언어와 관계없이 모델의 시작, 사용, 폐기 단계에 이르는 모든 단계에서 모델의 성능을 자동으로 모니터링합니다. 성능 벤치마킹 리포트는 모델의 스코어링 성과를 보여주고, 필수 표준에 대한 적합성 여부를 문서화합니다. 모델이 붕괴될 때는 이를 알려주는 경고가 생성되며, 모델이 서로 다른 부서에서 사용되는 경우에는 광범위한 추적, 검증, 감사 리포트를 생성합니다. 또한 다른 애플리케이션에서 사용할 챔피언 모델을 표시하여 보여줍니다. 지속적인 모니터링을 통해 사용자는 모델을 수정하거나 폐기할 시기를 알 수 있습니다.
내외부 변경사항을 반영하도록 모델을 조정하여 효율성 향상
변화하는 시장과 비즈니스 상황에 보조를 맞추기 위해 모델을 지속적으로 업데이트합니다. 새로운 데이터를 투입하여 기존 모델을 재분석하거나, 피처 엔지니어링 또는 새로운 데이터 요소를 사용하여 모델을 수정할 수도 있습니다. 모델 재학습은 효율성 향상을 위해 모델 파이프라인 처리 환경과 통합됩니다.
CI/CD 접근법으로 모델 라이프사이클을 자동화하여 시간과 리소스 절약
SAS Model Manager는 개방형 REST API를 사용하여 여러 환경과 툴, 애플리케이션의 통합을 가능하게 합니다. 또한 조직의 비즈니스 요구사항 및 프로세스와 일치하는 맞춤형 워크플로우를 생성하여 분석 라이프사이클을 자동화할 수 있습니다.
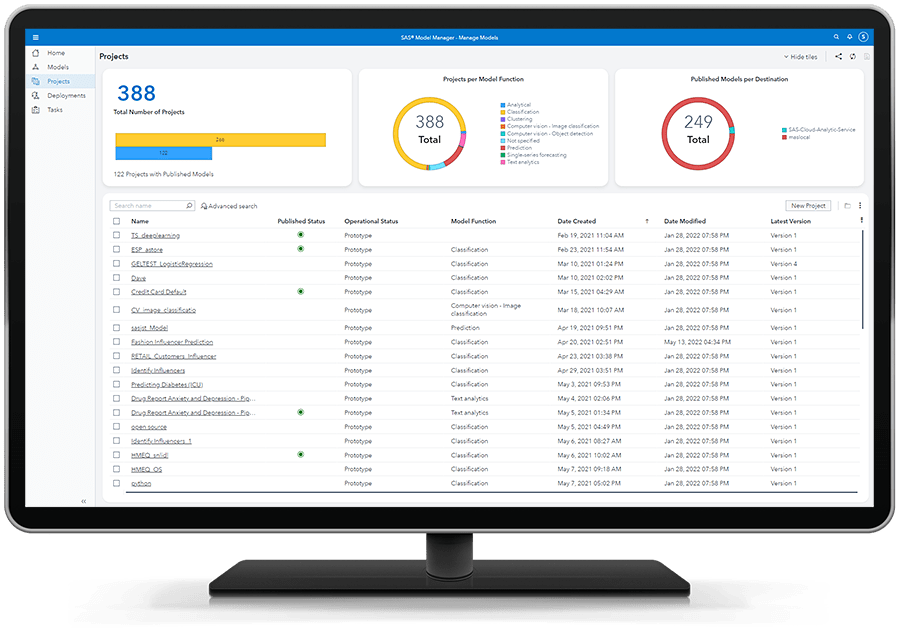
Free Trial
Try the latest SAS Viya capabilities.
Get a free, 14-day trial of SAS Viya, which includes SAS® Model Manager, as well as capabilities for the entire analytics life cycle.
SAS Model Manager를 지금 만나보세요!
주요 기능
모델 관리 프로세스를 자동화하는 웹 기반의 인터페이스로 모델 컬렉션 생성 및 관리 프로세스를 단순화합니다.
데이터 액세스, 준비 및 품질
임베디드 AI를 통해 셀프서비스 데이터 준비 기능을 제공하는 직관적인 인터페이스를 사용하여 데이터에 액세스하고, 프로파일링하고, 정제하고, 변환할 수 있습니다.
맞춤형 챗봇 생성
챗봇 기반 인사이트와 대화형 사용자 경험을 구현하기 위한 직관적인 로우코드 비주얼 인터페이스를 통해 자연어 챗봇을 맞춤형으로 생성 및 배포합니다.
데이터 시각화
셀프서비스 방식의 단일 인터페이스를 통해 데이터를 시각적으로 탐색하고, 스마트한 시각화와 대화형 리포트를 생성 및 공유합니다. 증강 분석 및 고급 기능이 인사이트를 가속화하고, 데이터에 숨겨진 이야기를 발견할 수 있도록 해줍니다.
검색 가능한 중앙집중식의 모델 리포지토리
중앙집중식의 안전한 웹 기반 리포지토리를 통해 분석 모델을 간편하게 관리할 수 있습니다. 사전 구축된 모델 라이프사이클 템플릿을 사용하면 프로젝트를 공동으로 관리할 수 있습니다.
맞춤형 워크플로우로 작업 자동화
문제 문(Problem Statement)의 생성, 모델 개발, 활용에 이르는 모델 라이프사이클 관리의 모든 단계에 대한 맞춤형 워크플로우를 정의하고 추적합니다.
한 번의 구축으로 어디든 배포
신속하고 자동화된 모델 배포 기능을 통해 비즈니스 프로세스에 모델을 배포할 수 있습니다. 단 몇 번의 클릭만으로 클라우드 또는 엣지에 일괄 또는 실시간으로 배포를 수행합니다.
REST API를 통한 프로그래밍 전용 상호작용
REST API를 사용하여 모델에 액세스하고, 비교 및 평가하고, 스코어링을 수행합니다.
클라우드 네이티브
SAS Viya의 아키텍처는 콤팩트하고, 클라우드 네이티브이며, 빠릅니다. SAS Cloud, 퍼블릭 클라우드, 프라이빗 클라우드 등 그 어떤 공급업체를 선택하든 클라우드 투자를 최대한 활용할 수 있습니다.
고객 성공 사례
SAS Model Manager로 더욱 스마트하게 일하는 고객