Real-world techniques for analyzing big data
Interview with author and professor Bart Baesens (Part 1)
If you have questions about the way big data and analytics are being applied today, Professor Bart Baesens is a good person to ask. Baesens is a professor at KU Leuven in Belgium and a lecturer at the University of Southampton (United Kingdom). He does extensive research on analytics, customer relationship management, web analytics, fraud detection and credit risk management. His findings have been published in well-known journals and presented at international conferences. He also regularly tutors, advises and provides consulting support to international firms with respect to their analytics and credit risk management strategy.
Baesens draws on all of these experiences in his new book, Analytics in a Big Data World: The Essential Guide to Data Science and Its Applications. We caught up with him recently to learn a little about what’s included in the book, trends he’s seeing with big data, and how organizations can modernize for analytics.
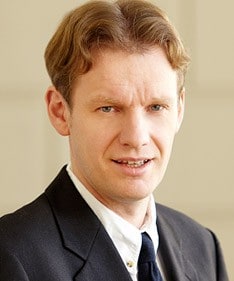
In order to fully leverage the power of analytics, two things are important: first, education of business users and secondly, visualization.
Bart Baesens
Professor and Author
KU Leuven in Belgium and
University of Southampton
Name two analytics techniques that provide the most value for analyzing big data in business environments.
Bart Baesens: Logistic regression has been the most valuable method traditionally, and social network analysis could be the most valuable technique in the future. Let me explain both in more detail.
Logistic regression has long been a popular analytical technique for doing classification. Example classification exercises include credit scoring, fraud detection, churn prediction and response modeling. In my research and consulting activities, I have found that logistic regression is a powerful technique with excellent explanation facilities that clarify its success.
I would guess every person is scored at least three times every day using a logistic regression model: once in a behavioral scorecard at your bank to predict whether you are going to default or not in the next 12 months for each of your credit products, once by your telco operator to predict whether you are going to leave them or not in the next three months, and once by your credit card provider each time you use your credit card.
Looking forward, I expect a lot of value from social network analytics. When I say social networks, I am not only referring to social media sites, such as Facebook, Twitter and LinkedIn. In fact, I am referring to any network of nodes (such as customers or companies) connected in a certain way [see image to the right].
For example, in a telco setting, the network could be built based upon call detail records data, where the nodes represent customers and the edges the phone connections between them. In a fraud detection setting, the nodes could be bank accounts and the edges money transfers between them.
Throughout our recent research with various companies, we have found significant added value from using social network analytics in churn prediction and fraud detection, compared to the traditional ways of doing analytics in both these settings. That’s why I also spent an entire chapter in my book on the topic of social network analytics.
What visual analytics techniques are most useful for big data? How does seeing and touching big data with visual analytics make it more approachable for average business users?
Baesens: Currently, there is a huge gap between analytics and business users. In order to fully leverage the power of analytics, two things are important: first, education of business users and secondly, visualization.
Without proper visualization aids, analytical projects are doomed to fail! Although visualization has been very popular during data pre-processing, we see it gaining importance during post-processing. Visualization encompasses both the representation of the analytical model itself as well as the accompanying reports needed for model monitoring, benchmarking and back testing.
Popular and user-friendly visualization mechanisms frequently adopted in model representation are decision trees and traffic light indicator approaches. For model monitoring, we see online analytical processing, multidimensional data analysis and digital dashboards becoming popular to monitor and analyze the various model KPIs online and in real time, as discussed in a separate chapter in my book.
Organizations are modernizing for big data in different ways, from cloud to grid to in-memory. What is your advice for IT organizations that haven’t made the leap to modernize yet but would like to?
Baesens: Big data and analytics are disruptive technologies, and they should be introduced in a wise manner. A couple of things are important here. The first thing I would say is to make sure you have a proper change management strategy to make the transition smooth. It makes no sense to buy extensive toolsets without being capable to use them. As described in my book, analytics is a step-by-step project and one should carefully consider each step before being successful. Also, I believe the demand for big data and analytics should come from the business, because that is where it’s going to end up being used.
The next important thing is education. Educating all stakeholders and decision makers by making them aware of what analytics can or cannot do, and describing the key challenges involved, is very important. This will make sure that expectations are properly set at the start of the analytics project.
Throughout the past few years, I have had a successful (and pleasant) experience teaching analytics concepts to business leaders through various Business Knowledge Series courses. We see a very strong need for this and are currently working on migrating some of our classes to e-learning to provide wider coverage.
Finally, corporate governance is also key. The whole big data exercise should be closely monitored and supervised by senior managers. I have seen quite a bit of big data and analytics projects fail because of lack of higher support in the organization. They should be actively involved throughout the whole exercise. In this context, we hear more and more about the need for a Chief Analytics Officer, which I definitely think is a good thing.
Read part 2 of our interview with Baesens, "The big effects of big data."
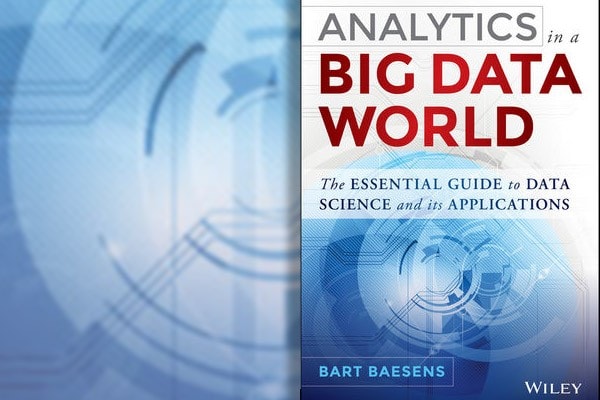
More Insights
- Analytics in a Big Data World draws on author Bart Baesens' expertise on the topics of big data, analytics and its applications in credit risk, marketing and fraud. Designed to be an accessible resource, this essential book does not include exhaustive coverage of all analytical techniques, but focuses on analytics techniques that really provide added value in business environments.
- Want to learn more about Professor Baesen's latest research? Follow his work at Data Mining Apps.
What is social network analysis?
Social networks are typically illustrated through a series of nodes and ties that show how the different nodes are connected. In this example, Bart Baesens is discussing the viral effect of churn. The nodes (or circles) are individual mobile customers, and the ties show how each person can influence other customers' decisions to switch mobile carriers.