Are you covering who you think you’re covering?
Healthcare beneficiary fraud can be a significant cost to health plans. Payers typically implement cost containment strategies through payment integrity efforts, which cover many types of improper payments. However, payers may not focus enough on beneficiary fraud, waste and abuse in public and private healthcare plans.
National health plans across the globe offer their beneficiaries no-cost or very low-cost services. Public health plans like Medicaid, administered by US states, also offer no-cost services to beneficiaries. This leaves little incentive for Medicaid beneficiaries to help contain costs.
Before paying a claim, payers need to be sure their beneficiaries are eligible. Payments for ineligible beneficiaries can be costly considering the costs of hospital emergency rooms and surgical procedures – up to thousands of dollars daily.
By comparison, one high-cost drug for one ineligible beneficiary may cost more than the monthly allotment of cash payments or food assistance for an entire family. A brief hospital stay can exceed the yearly family benefits for those other social benefit programs.
Spotting beneficiary fraud
Beneficiaries sometimes participate directly in fraud schemes related to medical services and pharmaceuticals. To spot collusive behaviors and abuse of prescription drug plans, payers must employ analytical techniques. Otherwise, they could overlook that what appears to be simple overutilization by beneficiaries is actually part of a bigger provider kickback fraud scheme.
A robust arsenal of advanced analytics applied to a broad range of data can help payers accurately detect and prevent beneficiary fraud. Such approaches include rules, anomaly detection, predictive modeling and link analysis. Here are key approaches to consider in flagging potential fraud, waste and abuse.
Analyze your existing data
Payers should analyze their existing data before seeking outside third-party data, which can be costly. Often, there are untapped structured and unstructured data sets that payers can use with beneficiary-eligible fraud analytics.
Start with matching techniques
Simple matching techniques can go a long way, but these require strong identity resolution processes. Such processes clean and organize data to find common people, places and metadata – like e-mail addresses, phone numbers, etc.
Use anomaly detection
Anomaly detection techniques, which look for outliers, can help identify beneficiary collusion with providers. Predictive models can help spot suspicious claims where the treatment pattern itself is an outlier. This could even include off-label drug prescribing.
Try link analysis
With link analysis, payers can spot unusual clusters of beneficiaries around certain providers and services. These techniques are exceptionally good at spotting beneficiary drug-seeking behaviors.
For example, any reviewer would find it suspicious when a husband and wife have matching pharmacy claims on the same day with matching diagnosis codes and doctor visits. These are signs beneficiaries are abusing the healthcare program or policy and possibly colluding in healthcare fraud.
Take advantage of text analytics and machine learning
These techniques assist with emerging healthcare fraud cases. Payers can use text analytics and machine learning, for example, to convert unstructured call center data into structured data for analysis.
Payers can also use text analytics to convert patient clinical records – and case management or care coordination notes – from PDF or other forms to structured data. This type of automation can deliver new results and encourage payers to implement more automation in work processes to help fight fraud, waste and abuse.
Contain costs with analytics
Beneficiary fraud in healthcare affects numerous areas, such as private health insurance, public health insurance, and programs like worker’s compensation or disability insurance. Advanced analytics is a trusted way to find hidden fraud schemes and beneficiary collusion with healthcare providers.
As data volumes increase exponentially, payers will be overwhelmed. And costs could spiral out of control without help from advanced analytic techniques that inevitably pay for themselves –perhaps many times over.
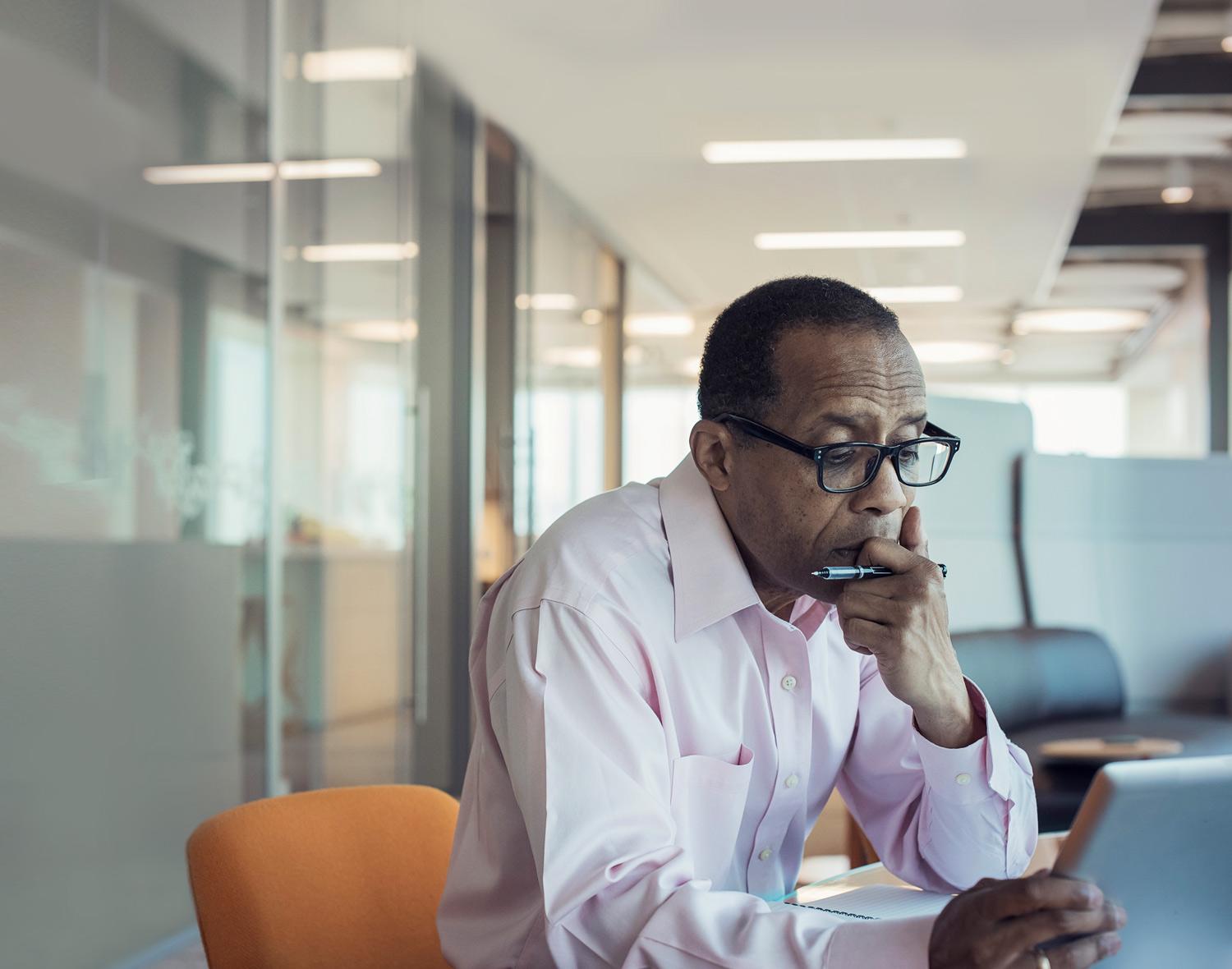