The “problem-solver” approach to data preparation for analytics
by David Loshin, President, Knowledge Integrity, Inc.
In many environments, the maturity of your reporting and business analytics functions depends on how effective you are at managing data before it’s time to analyze it. Traditional environments relied on a provisioning effort to conduct data preparation for analytics. After extracting data from source systems, the data landed at a staging area for cleansing, standardization and reorganization before loading it in a data warehouse.
Recently, there has been signification innovation in the evolution of end-user discovery and analysis tools. Often, these systems allow the analyst to bypass the traditional data warehouse by accessing the source data sets directly.
This is putting more data – and analysis of that data – in the hands of more people. This encourages “undirected analysis,” which doesn’t pose any significant problems; the analysts are free to point their tools at any (or all!) data sets, with the hope of identifying some nugget of actionable knowledge that can be exploited.
It’s important to ask the IT department to facilitate a problem-solver approach to data preparation by adjusting the methods by which data sets are made available.
However, it would be naïve to presume that many organizations are willing to allow a significant amount of “data-crunching” time to be spent on purely undirected discovery. Rather, data scientists have specific directions to solve particular types of business problems, such as analyzing:
- Global spend to identify opportunities for cost reduction.
- Logistics and facets of the supply chain to optimize the delivery channels.
- Customer interactions to increase customer lifetime value.
Different challenges have different data needs, but if the analysts need to use data from the original sources, it’s worth considering an alternate approach to the conventional means of data preparation. The data warehouse approach balances two key goals: organized data inclusion (a large amount of data is integrated into a single data platform), and objective presentation (data is managed in an abstract data model specifically suited for querying and reporting).
A new approach to data preparation for analytics
Does the data warehouse approach work in more modern, “built-to-suit” analytics? Maybe not, especially if data scientists go directly to the data – bypassing the data warehouse altogether. For data scientists, armed with analytics at their fingertips, let’s consider a rational, five-step approach to problem-solving.
- Clarify the question you want to answer.
- Identify the information necessary to answer the question.
- Determine what information is available and what is not available.
- Acquire the information that is not available.
- Solve the problem.
In this process, steps 2, 3, and 4 all deal with data assessment and acquisition – but in a way that is parametrically opposed to the data warehouse approach. First, the warehouse’s data inclusion is predefined, which means that the data that is not available at step 3 may not be immediately accessible from the warehouse in step 4. Second, the objectiveness of the warehouse’s data poses a barrier to creativity on the analyst’s behalf. In fact, this is why data discovery tools that don’t rely on the data warehouse are becoming more popular. By acquiring or accessing alternate data sources, the analyst can be more innovative in problem-solving!
Preparing data with the problem in mind
A problem-solver approach to data preparation for analytics lets the analyst decide what information needs to be integrated into the analysis platform, what transformations are to be done, and how the data is to be used. This approach differs from the conventional extract/transform/load cycle in three key ways:
- First, the determination of the data sources is done by the analyst based on data accessibility, not what the IT department has interpreted as a set of requirements.
- Second, the analyst is not constrained by the predefined transformations embedded in the data warehouse ETL processes.
- Third, the analyst decides the transformations and standardizations that are relevant for the analysis, not the IT department.
While it’s a departure from “standard operating procedure,” it’s important to ask the IT department to facilitate a problem-solver approach to data preparation by adjusting the methods by which data sets are made available. In particular, instead of loading all data into a data warehouse, IT can create an inventory or catalog of data assets that are available for consumption. And instead of applying a predefined set of data transformations, a data management center of excellence can provide a library of available transformations – and a services and rendering layer that an analyst can use for customized data preparation.
Both of these capabilities require some fundamental best practices and enterprise information management tools aside from the end-user discovery technology, such as:
- Metadata management as a framework for creating the data asset catalog and ensuring consistency in each data artifact’s use.
- Data integration and standardization tools that have an “easy-to-use” interface that can be employed by practitioner and analyst alike.
- Business rules-based data transformations that can be performed as part of a set of enterprise data services.
- Data federation and virtualization to enable access to virtual data sets whose storage footprint may span multiple sources.
- Event stream processing to enable acquisition of data streams as viable and usable data sources.
An evolving environment that encourages greater freedom for the data analyst community should not confine those analysts based on technology decisions for data preparation. Empowering the analysts with flexible tools for data preparation will help speed the time from the initial question to a practical, informed and data-driven decision.

David Loshin, president of Knowledge Integrity, Inc., is a recognized thought leader and expert consultant in the areas of data quality, master data management and business intelligence. David is a prolific author regarding data management best practices, via the expert channel at b-eye-network.com and numerous books, white papers, and web seminars on a variety of data management best practices.
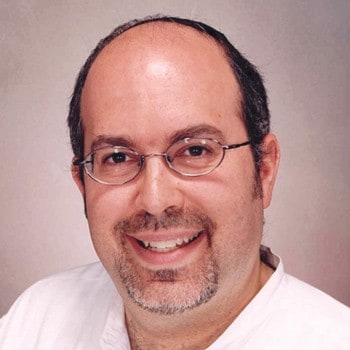
Get More Insights
Want more Insights from SAS? Subscribe to our Insights newsletter. Or check back often to get more insights on the topics you care about, including analytics, big data, data management, marketing, and risk & fraud.