Are you good at scoring?
Tips for reducing time-to-model while improving your results
By Anders Langgaard, Business Advisor, SAS
Credit scoring is the foundation for evaluating clients who apply for a loan (or other types of exposure for the bank). Building a credit scoring model is an exercise in statistics that typically involves big (or even huge) amounts of historical data.
As we saw during the COVID-19 pandemic, nontraditional data took on new significance (this data is sometimes called new data or alternative data). But at many banks, it can take six months or more from the moment you decide to build a new model until the model is deployed in the production environment.
As the world continues to change, the significance of model parameters changes with it. All the while the model is losing precision. Lower model precision, in turn, leads to erroneous borrower ratings, which can cause the bank to take on risky or unprofitable loans.
Credit scoring on the cloud
Learn how S-Bank, Finland's top retail bank, modernized with SAS Viya on Azure and gained access to improved visual tools and faster performance from running analytics closer to its data. Analytics from SAS led to faster loan processing times, while automated processes allowed for faster, more accurate decision-making.
A (business) case of shorter time-to-model
SAS ran a business case with a small American bank with total assets of $1 billion. The bank had, on average, credit losses of $25 million per year. Using credit scoring tools that enabled higher performance in the scoring process – including the model development phase – the bank cut its modeling cycle from four months to two months. In turn, it reduced losses by 5% – simply by using better credit scoring models earlier in the process.
But how can you shorten the model life cycle? First, let's look at the steps of a life cycle:
- Formulate a hypothesis.
- Prepare the data from which to build the model.
- Explore the data to ensure the quality is sufficient and to make sure the data contains the needed information.
- Transform the data. Most likely, some of the data will need to be transformed into useful variables.
- Build the model using statistical tools.
- Validate the model (that is, ensure that the model still performs on data that was not used for building the model).
- Deploy the model in production – perform the scoring of the clients.
- Evaluate and monitor the performance of the model.
Potential time thieves in the model life cycle
At many financial institutions, the analyst (the model developer) must ask the IT department for the data that's needed. The IT department then gathers the data from several sources and delivers it to the analyst – which could take two to four weeks. If the analyst finds that something is missing in the data, the process starts over.
A good way to achieve a leaner process would be to enable analysts to directly build their own data sets. You should also give analysts tools that make it quick and easy to test their hypotheses.
Examples include data visualization techniques and in-memory processing. This combination provides an easy view of the data. And with high-performance analytics, it delivers lightning-fast answers.
Automate and integrate
The steps you need to take from the moment you validate a model to the moment you can put it in production is a final time thief.
At many financial institutions, there is no link between the development environment and the production environment where the scoring takes place. This means that the model is often (manually) carried from the analyst back to IT, where the model is recoded to another programming language so that it can finally be implemented in production. This raises operational risks – including the risk of model coding errors. Additionally, it makes model validation and performance measurement difficult to perform.
It’s easy to see that streamlining the model development process will reduce losses and increase earnings. It will also reduce the institution's operational risk. The combination of these efforts will help validate the business case for investing in these improvements.
Considering alternative data sources?
One of the most important things that happens during the process of incorporating nontraditional information sources (sometimes called new data) is finding the business logic behind creating the variables. Each new variable must be checked for quality and a hypothesis needs to be formulated so that the additional data will add value to the result.
The modeling process is practically the same. After variables are created and validated, special attention must be paid to the ethics, stability and biases that the new sources of information can bring. At this point, information governance and model risk management become deeply relevant.
For many firms, updating the model process to use alternative data in their scoring models has been on the horizon as an opportunity for some time. Now, circumstances make it a necessity.
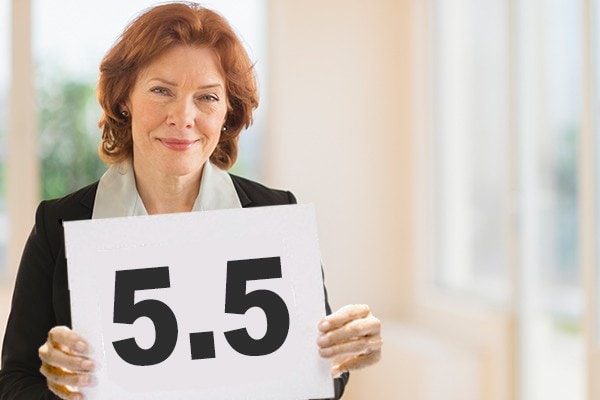
Learn more
- Download our white paper: Outrunning risk with cloud
- Read about: Credit risk management from SAS
Get More Insights
Want more Insights from SAS? Subscribe to our Insights newsletter. Or check back often to get more insights on the topics you care about, including analytics, big data, data management, marketing, and risk & fraud.