A framework for analytics governance
5 steps to making sure your analytics projects are useful, secure and repeatable
By Alison Bolen, SAS Insights Editor
In almost every industry, the use of analytics is exploding. You now have access to more data than ever before and, thanks to low-cost storage options like Hadoop, you can store it all at an affordable price. Add to that a growing list of user-friendly technologies for managing, accessing and analyzing data – and it’s no wonder the use of analytics has spread to all corners of the organization.
It’s not unusual to see decision makers in the finance department visualizing billions of rows of risk data while analysts in marketing deploy open source models to identify customers for a new product offer. Meanwhile, data scientists in product development are using public data sources to anticipate the needs of customers over the next five years.
How can you be sure that all of these efforts are accurate, aligned and worthwhile? How do you know the analytics processes are being deployed, repurposed and secured as efficiently as possible?
Without analytics governance, you don’t. Even if you have a handle on your data management and data governance policies, you still need to consider the benefits of putting policies and procedures around the analytics process as well.
Data governance ensures you have structures and policies around the use of data in your organization, and analytics governance applies the same level of scrutiny to the way analytics projects are implemented and deployed.
It’s not enough to run analytics, get a decision and you’re done. There’s more to it. You put all the time into data preparation and model building, you need to make sure the resulting analysis is something you can complete and repeat.
Mike Frost
Director, Product Management
SAS
Governance is the critical last mile for analytics, explains Mike Frost, Director of Product Management at SAS. “It’s not enough to run analytics, get a decision and you’re done. There’s more to it. You put all the time into data preparation and model building, you need to make sure the resulting analysis is something you can complete and repeat.”
Without analytics governance, you are opening your organization up to a laundry list of risks, ranging from competitive sabotage of your models to the common problem of data models that are built but never used.
“Companies must develop not only analytic skill sets but also the enabling processes that help analytics transcend interesting to actually driving business action and outcomes,” says Kimberly Nevala, Director of Business Strategies for SAS Best Practices.
And what about security? “You have all these models, algorithms and data pieces being created,” explains Frost. “When you start assessing the risk of doing scoring and modeling, you need to evaluate what kinds of algorithms are in your network. Could these models be a Trojan horse – an easy way for someone to mess with your decision-making capabilities? Organizations need to have something to oversee all the data scientists writing algorithms that are core to the business.”
To that end, here are five steps to mitigate the potential risks associated with the decentralized use of analytics.
- Develop an analytics governance board that works to align analytics projects to business goals. Make sure the team includes an executive sponsor and representatives from all organizations that are using analytics. This team will establish procedures and guidelines to ensure the analytic agenda aligns with organizational objectives and each project has a clear goal and measures for success.
- Track and coordinate analytics projects with the governance board acting as the coordinator for all analytics activity. The board will not only keep track of analytics projects but communicate to the rest of the organization about what data is being analyzed and how it is being used. In addition to tracking where analytics are coming from, this step involves building an audit trail that tracks the libraries and metadata that go into building your models. The audit process will help prevent duplication of efforts and a proliferation of models that are not fully implemented and used.
- Deploy models into a production environment that decision makers know how to use. Where possible, embed models into systems where automatic decisions can be made. This will help ensure that your analytics efforts are being put to good use around the organization.
- Measure results and continuously update models to keep pace with changing business conditions. This should be done consistently across all analytics projects with regular review and updates, to ensure your models are always current and performing at their best.
- Develop strict security guidelines for your analytics systems and processes that keep your results safe and adhere to industry regulations. Tracing the history and histology of those models will help address security concerns better and with a higher degree of confidence. Again, the analytics governance board will play a crucial role in developing and enforcing appropriate security guidelines.
Analytics governance is not meant to slow down or hinder creativity in the analytics process. Rather, the goal is to ensure creativity is focused and harnessed for the good of the organization.
“Done right, analytic governance balances the need for exploration and experimentation with the need for insights that can be deployed to help the business,” concludes Nevala.
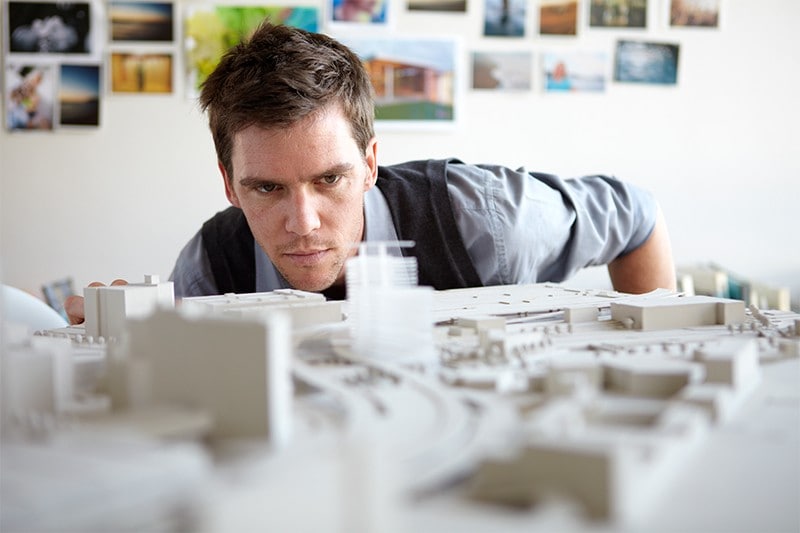
What to Read Next
Discover how ModelOps can improve your analytics governance.
Get More Insights
Want more Insights from SAS? Subscribe to our Insights newsletter. Or check back often to get more insights on the topics you care about, including analytics, big data, data management, marketing, and risk & fraud.