Business Analytics Series – Part 1 of 3: Reporting with Data is not the same as making Decisions with Data
“Thank you for spending these past few weeks on this great report. I can see what’s been happening to the business over the last year, but what does it tell me about how we’re currently doing, and what we should be doing to continue growing?”
Sound familiar? You’re not alone.
In my role as an analyst, I talk to executives on a regular basis about the challenges that they experience in their respective organizations. While the unique challenges for each are often quite varied, there is one thing that I consistently hear: they aren’t convinced that they are making the most of their data. Sure, they’re often given reports based on last month’s numbers, or shown trending analysis depicting year-over-year changes, but when it comes to translating that into a tangible, measured action, they are often left with the notion that they are still making a bit of a leap of faith.
Now don’t get me wrong, those reports are still extremely valuable—whether housed in Excel (as so many are), or in some other Business Intelligence (BI) system—historical data has its place in the enterprise. That said, to turn those reports into insights, to take that data and make not only informed and educated decisions, but ones with reasonably predictable outcomes based on advanced pattern recognition and regression requires a new approach, and a new class of tools: Business Analytics (BA).
What are Business Intelligence and Business Analytics, and how are they different?
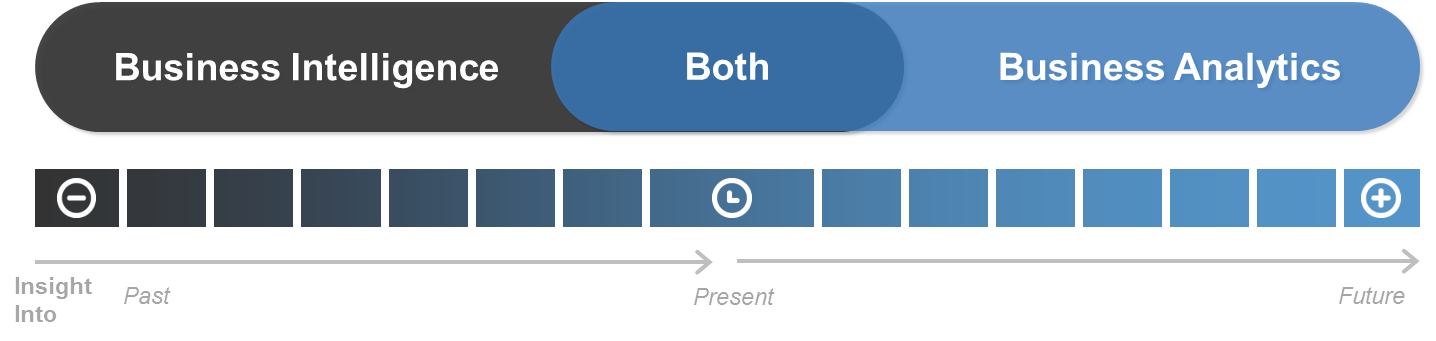
Business Intelligence: BI is heavily focused on observing historical and present data to create reports and dashboards that inform a wide range of users on past and current affairs. Surprisingly, even though BI has been such a hot topic of late, the overall maturity of Business Intelligence technology in organizations is still relatively low, as evidenced by the amount of data still generated, stored, and analyzed in Excel.
Business Analytics: BA is a natural next step to BI, with a focus on evaluating and assessing the present and future of the organization using regressions, trends, and forecasts. These advanced analyses allow for predictive modelling, what-if scenarios/cause and effect impacts, and incorporate a wider variety of data sources, both structured and un-structured, compared to Business Intelligence.
Both of these tools have their place in a company. You can’t make decisions without understanding the past, but you also can’t make decisions without having some insight into the future.
What are the key benefits of Business Analytics tools?
It’s easy to say that Analytics provides insight into the future, but relating that to specific business benefits can be tricky, especially if the explanation is coming from a technical (IT) side, rather than a functional (business) side. To illustrate, we can take a very common scenario for most organizations and look at both BI-driven, and BA-driven approaches: monthly sales targets.
The typical sales manager will have a pre-configured report to review his or her team’s sales to date on a daily basis, monitoring progress against the monthly target. They may even have a rolling “daily sales need” (possibly also auto-generated from the data) for each remaining day depending on how close, or far, the team is from that target. This is a typical way of using BI to provide information on “how much more we need to sell each day to hit the target”.
Now let’s look at the same situation using BA instead. This sales manager has the same reports (remember, BA is built off of a BI foundation), but instead of relying on the rolling calculation of “how much more we need to sell each day to hit the target”, this manager has a different approach.
Using an analytics engine, the manager is provided a predicted monthly outcome based not only on sales to date, but also previous years’ sales for the given month, an expected renewal and/or conversion rate, and a predicted average selling price. With these analytics tools at his/her disposal, the manager can very quickly (and without the need of IT intervention) compare scenarios where they might offer discounts of 5, 10, or 15% to ease that price sensitivity, and watch as the algorithms calculate expected outcomes based on that change. The tool indicates that a 5% discount is not enough, a 15% discount would increase conversions, but the drop in ASP would be too great, but a 10% discount will have a similar effect on conversion, without a major hit to ASP, bringing the team’s monthly projection in line with the target.
So why are so many organizations still only using BI and reporting?
Based on the benefits companies can reap from BA you may be wondering: well, why aren’t companies transitioning to Business Analytics?
The reality is that deriving great insights through Analytics is predicated on things like good data quality, robust integrations, and processing and storage capabilities (to say nothing of the cultural and user-experience components) that not every organization has at the moment. Before making the leap head-first into an Analytics initiative, I always recommend that both business and IT come to agreement on the benefits, risks, costs, and implications of that initiative, and the best format for that is the business case.
Stay tuned for Part Two of Three in our Business Analytics Blog Series where we will cover how your organization can build a business case for Business Analytics and join the shift towards BA
About the Author:
Daniel Ko has been in the information management field for over 10 years and he has a solid understanding of BI, BI strategies, analytics, data integration, ETL, data quality, data hygiene, data architecture, data warehousing, datamarts, GIS (Geographic Information Systems), Big Data, and Master Data Management (MDM). He has assumed the roles of Subject Matter Expert (SME), Project Technical Lead, Project/BI/Data Warehouse Architect, Project Assessor, Data Analyst, and Business Analyst in various projects and positions.
Prior to joining Info-Tech Research Group, Daniel worked at Project X Consulting as a Senior Technical Consultant and helped telecommunication, insurance, and healthcare clients on their information projects. Formerly he served as a BI Team Lead at Adesa, coaching the BI team and helping external and internal clients to leverage data and data management technologies. Earlier in his career, Daniel worked as a Data Warehouse Analyst at TELUS Mobility and enabled the business to uncover knowledge and insights. Daniel also worked in the automotive and utility industries.
Daniel received his B. Sc. from the University of Toronto. His major is Molecular Genetics with a number of courses in GIS (Geographic Information Systems).
Daniel is MicroStrategy CPD, CDD, CRD, and Oracle 9i DBA-OCP certified. Daniel is also a certified PMP.