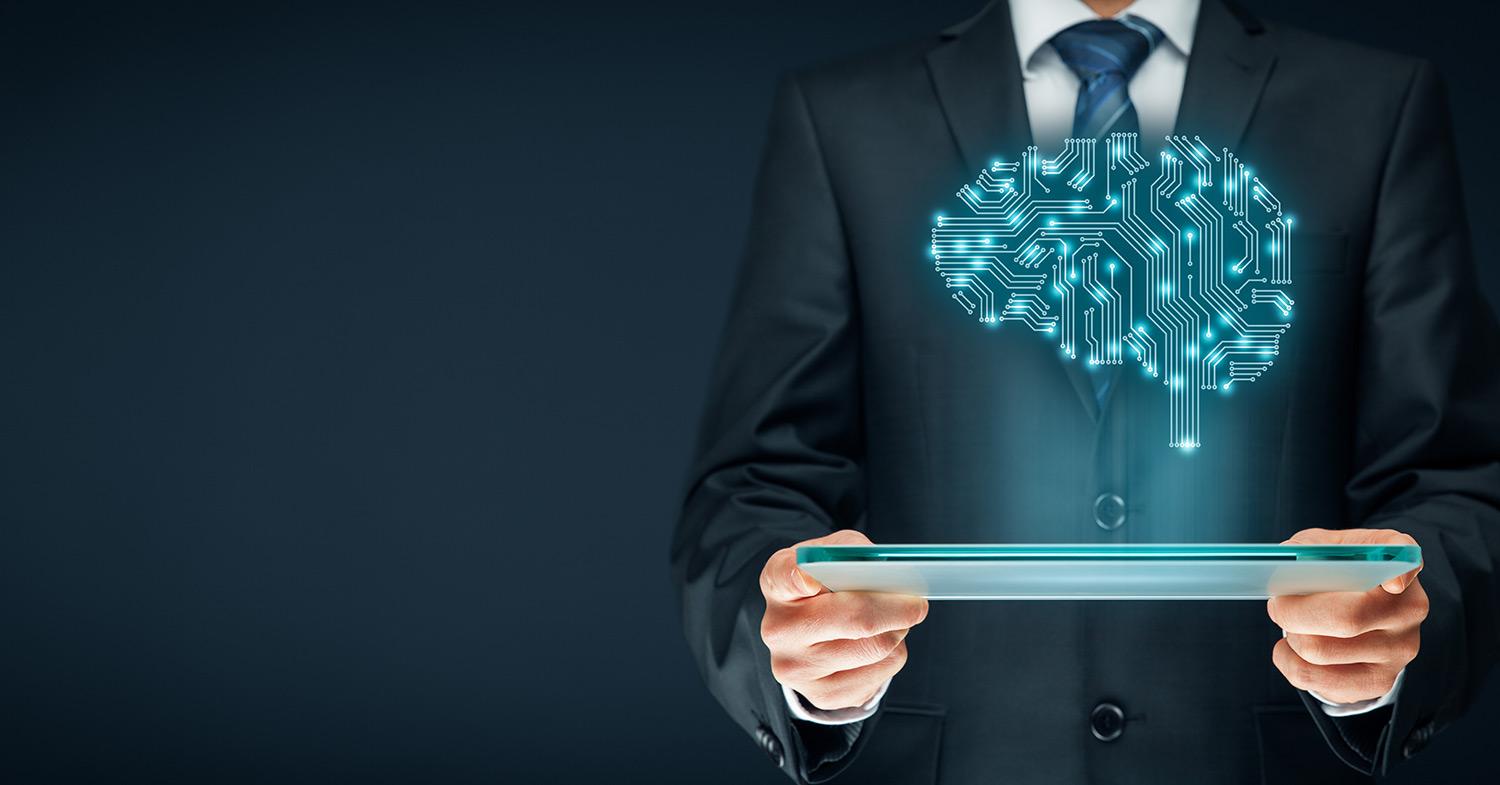
Real World AI
Turning business problems into prediction problems—and solving them
Steve Holder, National Strategy Executive Analytic & AI at SAS
That item you need just arrived. Enjoy. Never mind that you hadn’t gotten around actually to ordering it online.
The popular vision of the future of artificial intelligence—autonomous machines mimicking human behavior and thought, becoming sentient beings—may be in the cards, but that’s far from certain and, for business today this vision is less relevant. More likely scenarios are those like the one outlined by Ajay Agrawal at the recent AI in the Real World event hosted by SAS in Toronto: When Amazon has gathered enough detailed information, run it through their algorithms and applied their models, they’ll be able to ship you what you want before you order it, pre-empting your purchase from another supplier. Amazon will need to find a threshold that balances the incremental profit against the cost to process unwanted items and returns.
Since Amazon has already applied for a patent on “anticipatory shipping,” we may see that scenario from Amazon sooner rather than later.
Agrawal, an economics professor at University of Toronto’s Rotman School of Management and co-founder of its machine-learning-focused Creative Destruction Labs, a pre-seed incubator, likes to substitute the words “cheap prediction” for “artificial intelligence.” Through that lens, it’s easier to disentangle the real promise of AI—lowered prediction cost and lower error rates that complement increasingly valuable human judgment. This model for AI is easy to discern from the volume of analytical work being done in organizations today and applying “cheap prediction” means solve more business problems.
Artificial Intelligence in the Real World:
Artificial intelligence in the real world is making an impact. SAS spoke with a few of the top AI thought leaders: Professor Ajay Agrawal, Forrester Analyst, Mike Gualtieri, Head of Accenture AI, Jodie Wallis, and Head of Deloitte AI (Omnia) Shelby Austin to learn what they had to say about it.
- 2x
- 1.75x
- 1.5x
- 1.25x
- 1x, selected
- 0.75x
- 0.5x
- Chapters
- descriptions off, selected
- captions settings, opens captions settings dialog
- captions off, selected
This is a modal window.
The Video Cloud video was not found.
Beginning of dialog window. Escape will cancel and close the window.
End of dialog window.
This is a modal window. This modal can be closed by pressing the Escape key or activating the close button.
This is a modal window. This modal can be closed by pressing the Escape key or activating the close button.
This is a modal window. This modal can be closed by pressing the Escape key or activating the close button.
This is a modal window. This modal can be closed by pressing the Escape key or activating the close button.

Those prediction problems are more ubiquitous than you may think. Take the hiring process for example, says Agrawal. It’s traditionally seen as a “soft” science, one that’s largely dependent on impression and intuition, and not suitable for automation. Like it or not some believe it’s the kind of process where AI excels: predicting outcomes based on a wide range of inputs. It’s not just about the candidates’ resumes or personality. AI could consider internal employees who might be candidates for the position. Assess whether those employees are flight risks because of their attractiveness to competitors and should be retained with an offer of promotion and offer advice to help narrow the field.
Pragmatic AI
There’s pure AI and there’s pragmatic AI, says Mike Gualtieri, vice-president and principal analyst for AI at Forrester Research, who also presented at the AI in the Real World event hosted by SAS in Toronto. Pure AI—mimicry of human intelligence—is a hypothetical that may be achieved or may not. Pragmatic AI—machine learning, natural language processing, modeling, use of automation and adaptation —is where business advantage lies. A lot of effort is wasted on analytics that aren’t aligned to a business outcome. Identify high-value business processes that are data-rich and apply AI there.
Algorithms get all the attention, Gualtieri told the conference, but the entire model falls apart without the data. For all the technological advances in the algorithms that AI is based on, without good data it’s still garbage in, garbage out.
AI is a spectrum of processes for ingesting, contextualizing, recognizing and understanding data. It’s built around an intersection of data and business process that defines value. It’s not magic, a crystal ball, a cure-all for any business problem. AI takes analytics, amplifies them, and brings them into the real world. AI can help organizations acquire and categorize information, adapt to changing environments, deliver faster time to insight and in some cases automate business decisions.
AI Momentum, Maturity and Models for Success
A report based on a global executive survey
Making Connections
This real-world integration of AI requires technologies that mimic a human’s experience in the physical world: natural language processing to hear and speak to the world, processing of structured and unstructured input, logic and algorithms to reinforce learning of environments, video interpretation or robotic processes to interact with objects.
Enter the application programming interface (API). The API model—using a set of protocols and libraries to connect software components—has been around for many years. The use of APIs has allowed software developers open and standardized ways to interact with complex, disparate and often proprietary systems in a common and consistent way. SAS has used the representational state (RESTful) architecture to embed our AI algorithms in a variety of programming languages—Python, R, Lua, Java—and use open source models from TensorFlow, Caffee and others. Programmers can use the programming syntax they know and deploy the AI system into the organization’s enterprise architecture.
Analytics and Maturity
What’s the connection between analytics and AI? It’s a common question. There’s a relationship between the maturity of AI initiatives and the role that analytics plays a foundation for successful AI.
In a recent Forbes survey, nearly half (46 percent) of AI adopters overall said their organization has fully deployed AI, either in one or multiple use cases. Other adopters are still in the experimenting and prototyping phase. When you look at the role of analytics in AI projects and the associated maturity the impact is significant. Among organizations still in development with their AI initiatives, only 21% saw analytics having a critical or major role. In contrast companies with mature AI projects, 77% saw analytics having a critical or major role. These numbers show that to be successful with deployed AI, analytics need to be a central and integral part of your AI strategy. Speaking of an AI strategy, what do you need to consider in order to move from AI hype to pragmatic AI? There are four primary considerations:
Explain. What is the initiative doing? Knowing how to state the goal and reason for an AI initiative helps avoid wasted time on the irrelevant. Organizations also must create transparency in the AI system--being able to explain why a decision was made helps manage the impact of AI to ensure trust.
Control. This isn’t about access. It’s about oversight—understanding what the system is doing to ensure it’s doing the right thing, not just doing things right. AI and analytics are driven by data and as a result the efficiency and relevance can decay or become biased. The right answer to the wrong question at the wrong time can be dangerous. Having a controlled system in place that knows what the AI system is doing, and where and why it made a decision, helps organizations ensure AI is working for them, not the other way around.
Scale. Doing something once is easier than doing something hundreds of times. AI systems need to be able to scale from concept, to experimental application and be able to move to full-fledged deployment while minimizing duplication of effort and resources. Is your infrastructure—not just computing infrastructure, but business process and governance—ready for the impact of multiple AI system rollouts?
Choice. Expanding beyond the scope of technologists to the realm of business users requires multiple approaches to interact with AI systems. While some people are comfortable building an AI system by writing code and integrating a variety of open APIs, there are many individuals who don’t have the desire or skills to build it from scratch. Organizations need the ability create democratized AI that allows business users to become savvy with AI approaches without writing code, while at the same time enabling the technologists to build.
The SAS approach to AI is to augment human efforts through algorithms and automation. Our technology helps organizations:
- Address the diversity of AI capabilities and users to help organizations democratize AI, regardless of skill level or language preference.
- Scale your AI initiatives from concept to deployment by maximizing performance and minimizing effort and infrastructure duplication.
- Future proof against the changing AI ecosystem and create consistency in your AI outcome for all users.
- Deliver AI in a trusted and transparent way to help your organization deliver on your business objectives without having to worry about building an AI engineering team.
If that resonates with your company, get in touch, we can help draw your AI roadmap together.
And by the way, a package arrived for you …
About the Author
As the Strategy Executive at SAS Canada Steve Holder is responsible for creating and driving the SAS solution strategy with our Canadian customers. A key part of this is providing thought leadership for the SAS Analytics, AI, Big Data and Cloud portfolios including Open Source integration. A Canadian AI evangelist Steve has seen first-hand how the use of AI, analytics and data can help customers solve business problems; make the best decisions possible and unearth new opportunities. Steve’s passion is making technology make sense for everyone regardless of their technical skillset. Steve tweets at @holdersdh and can be emailed at steve.holder@sas.com.
Recommended reading
-
Understanding digital twin technologyLearn how digital twin technology can change industries from health care to manufacturing, and shape the future of data modeling and process improvements.
-
A data scientist’s views on data literacyData literacy is a social imperative – and understanding data and data analysis is critical for being a responsible citizen. Get a data scientist and teacher's perspective on the value of having foundational knowledge so you can more easily tell data facts from data fiction.
-
The 5 new rules of retailThere is good news for retailers. Analytics can help overcome some of the effects of disruption, allowing retailers to move from long-term seasonal forecasting to more agile planning.
Ready to subscribe to Insights now?
SAS® Viya™
Make analytics accessible to everyone and bridge the talent gap in your organization