Applying machine learning to IoT data
Can machine learning bring a concrete aspect to IoT projects?
By Mika Tanskanen, Manufacturing Industry Consultant, SAS Finland
The idea of an intelligent, independently learning machine has fascinated humans for decades. I remember how the concept became reality for me after I purchased my first computer, the legendary Commodore VIC-20.
When I demonstrated the computer for my grandmother, she began by saying, "Could you ask that machine ... ?"
She was clearly ahead of her time. Not to downplay the capability of the VIC-20, but at the time, it would have been premature to discuss, for example, neurorobotics as a part of everyday life in eastern Finland, where I grew up.
This was an era far before Apple's Siri, or any other assistants based on speech recognition technology. Back then, there was no Internet to connect computers to. And even my compact cassette recorder had more storage space.
The storage capacity and other capabilities of modern technologies are light-years away from the days of my youth. And technology has become affordable for nearly everyone.
The philosophy behind machine learning is to automate the creation of analytical models in order to enable algorithms to learn continuously with the help of available data.
From VIC-20 to machine learning
Artificial intelligence and machine learning are not novelty innovations. As early as 1959, Arthur Samuel defined the concept of machine learning as the ability of computers to learn to function in ways that they were not specifically programmed to do. Of course, the timeline from definition to implementation in everyday life can be a long one. Today, many factors have come together to make machine learning a reality, including large data sources that are great for learning, increased computational power for processing information in split seconds, and algorithms that have become more and more reliable.
Machine learning can be applied in cases where the desired outcome is known (guided learning), or the data is not known beforehand (unguided learning), or the learning is the result of interaction between a model and the environment (reinforcement learning).
The philosophy behind machine learning is to automate the creation of analytical models in order to enable algorithms to learn continuously with the help of available data.
Continuously evolving models produce increasingly positive results, reducing the need for human interaction. These evolved models can be used to automatically produce reliable and repeatable decisions.
Examples of everyday application of machine learning include recommendations made by online services (Amazon, Netflix) or automatic credit ratings by banks.
How far has machine learning evolved in concrete terms? While it’s not possible to tell your car to take you to work, that day is coming soon.
Google's self-driving vehicles are hardly news to anyone today. By combining the advanced features of modern cars (speech recognition, adaptive cruise control, lane assistant, navigator and parking assistants), we are close to a completely independent operating vehicle.
One of the most significant impediments to machine learning is legislation, as today’s laws need to catch up with the technology that’s already available. We probably won’t see the day where a machine defends itself in court, but we do need to consider how much power we’re willing to give machines that can make independent decisions.
Machine learning plus IoT
Machine learning has experienced a boost in popularity among industrial companies thanks to the hype surrounding the Internet of Things (IoT). Many companies are already designating IoT as a strategically significant area, while others have kicked off pilot projects to map the potential of IoT in business operations.
As a result, nearly every IT vendor is suddenly announcing IoT platforms and consulting services.
But achieving financial benefits through IoT is not easy. The lack of concrete objectives is disconcerting. The advancement of digitization and IoT places new prerequisites on both buyers and sellers. Many businesses have failed to clearly determine what areas will change with the implementation of an IoT strategy.
In other words, clearly defined, concrete intermediary objectives are missing. For example, industrial companies produce a massive amount of data on a daily basis. However, by and large, companies fail to systematically collect, store, analyze and use such data to improve process efficiency or meet other goals.
Furthermore, not many vendors are able to establish, in concrete terms, to the client how to prudently create positive impact on business operations with IoT solutions. Simply the promise of a cloud-based IoT platform is not enough.
According to Gartner, Finland is headed toward a trap where discussion about IoT revolves around technical terminology instead of business goals. Clients need to adopt innovative ideas and have the courage to embrace change. Conversely, vendors need to improve their skills in describing, in more concrete terms, what companies can realize through the use of IoT, and be willing to help identify business opportunities and develop realistic plans.
If a vendor offers a solution based on a single analytical method for basically all problems, alarm bells should be ringing.
Finding answers in IoT data
A while back, my colleague was asked to assist a company in the energy sector. The company's new facility was experiencing severe production difficulties resulting from a critical turbine failure.
A third party had been hired to solve the matter. After around six months of work, a team of four consultants using only path analysis had made little progress.
The facility had produced plenty of machine, maintenance and process data, as well as time-stamped weather information. Despite all of this available data, no one was able to refine it into useful information.
To get past that standstill, we helped the organization add an analytical component. Once all of the data was refined via analytics, the underlying problems were found to be related mainly to oxygen feed optimization during the production process. The facility has not had any major issues since.
This is a prime example of how machine learning can be used to achieve even higher levels of efficiency. With the right algorithms, the system can be gradually taught to recognize any internal and external production-related factors, optimize the use of consumables, and improve the efficiency of the entire production process.
This is just one of many great examples of IoT in practice. For more, you can read a thorough study about three organizations analyzing IoT data.
This article was originally published in Finnish on the Hidden Insights blog.
Mika Tanskanen works with Finnish industrial companies, collaborating with clients in finding ways to use analytics for the advancement of business operations. He is also an absolute mechanics enthusiast, spending his spare time, for example, maintaining and customizing his 40-year-old motorbike.
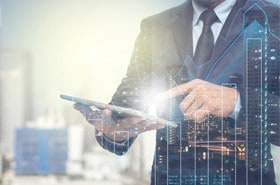
What to Read Next
Get More Insights
Want more Insights from SAS? Subscribe to our newsletter. Or check back often to get more insights on the topics you care about, including analytics, big data, data management, marketing, and risk & fraud.