Using Hadoop as an analytics catalyst
Reprinted with permission from CIOReview magazine
By Paul Kent, VP of Big Data, SAS
Your Chief Marketing Officer is pestering you to link social media data to sales, the risk team wants to run more data through high-speed analytics to prevent fraud before it occurs and the supply chain gurus are trying to manage an avalanche of sensor data that could reshape how raw materials make it to your plants.
Sound familiar?
If you are getting requests like these you’re also getting feedback that Hadoop will take care of all the Big Data challenges listed above. We don’t blame you if, instead, you think of Hadoop as a cheap, overly hyped storage solution that won’t take care of all those problems. This, however, isn’t another story about the glories of Hadoop. We’re going to explore some examples of organizations using Hadoop to drive innovation.
Hadoop has gained a deserved reputation as a solution to thorny data problems. Whether the information comes from financial trades, tweets, retail transactions or social media interactions—this data was too expensive to process, store and analyze in the pre-Hadoop days.
Hadoop’s ability to collect data and distribute it across multiple nodes on servers, and then process a subset of the data in parallel, mimics the actions of Grid Computing, but at a far more reasonable cost, and with far greater accessibility to every enterprise.
Hadoop allows for shortcuts that allow enterprises to process and analyze huge amounts of data with fewer steps, quickly and at lower cost.
A sound Hadoop strategy
Unfortunately, organizations fall into the trap of using Hadoop like a cut-rate storage unit that you shovel content into–and then forget about.
Hadoop is a natural technology to support an analytics platform. Its parallel processing capabilities make it a powerful and blazing fast engine for analytics.
With Hadoop and analytics software, you can easily build predictive models—using data not only to see what happened but what is likely to occur and what’s the best course of action.
Five years ago, these model factories were an idea in need of cheaper processing capability and more robust analytics. Today, they are a reality:
- AT&T is using Hadoop as an analytics platform for predicting when a customer may be about to defect and determining the best ways to intervene.
- Cisco is using Hadoop to build 30,000 propensity-to-purchase models per quarter. The company’s depth and breadth of product requires this number of models. Without Hadoop, this would be too expensive and so time-consuming the models would be out-of-date before they can be deployed.
- A large financial services company is using Hadoop to construct a massive database for all its customer cross-sell, upsell and attrition prevention activities.
- Facebook, Yahoo, Netflix, LinkedIn, Amazon and Twitter are all known to be big Hadoop users. In fact, most of these don’t just use Hadoop; Hadoop is ultimately what runs their businesses. When you post on Facebook, for example, you’re dealing with a social networking site driven by a Hadoop based back end.
Hadoop and the new analytics culture
Hadoop lets you develop analytical models using all data, not just a subset. You can run frequent modeling iterations and quickly get answers to all sorts of questions you never thought to ask or had time to ask. Sometimes data collected for different purposes can be reused in innovative ways.
A commercial real estate company in Japan figured out that the elevators in its buildings kept log files. By running analytics on the logs, the company determined which floors were seeing more activity. The company realized that this information could provide an indication of which tenants were experiencing strong business and might be in the market for more space, and which might be slow and a risk for moving.
One of the big hurdles in using lots of data is not being stuck in the mode of constantly looking back in time. Hadoop allows data-driven decision-making in near real time.
For example, a retailer discovered that the digital images of security cameras in parking lots could be analyzed in real time to make stores smarter about when it’s about to get busy and deploying employees accordingly.
Hadoop and big data management
Hadoop combined with analytics fundamentally changes the nature of data processing. Traditional methods involving extracting data from enterprise application like CRM and ERP systems involve multiple processes. Hadoop allows for shortcuts that allow enterprises to process and analyze huge amounts of data with fewer steps, quickly and at lower cost.
Paired with business user friendly analytics, a marketing director, for example, no longer has to wait for a static report on a subset of data that may tell something about regional difference in product buying patterns; he or she can analyze much larger volumes and types of data to glean insights that include all sorts of factors, not just regional ones.
Getting the most out of Hadoop
One stumbling block is how to introduce Hadoop without a wholesale configuration of your IT architecture. It’s a realistic concern. Companies have invested substantial sums in their existing data infrastructures—from software licenses to hiring, training and retaining the right technical people at a time when those skills are tight. No one wants to rip out their current infrastructure.
The good news is they don’t have to. Products and services exist to create seamless and transparent access to Hadoop. Some of these tools are highly visual and interactive, making it simpler to gain insights and discover trends.
New interactive programming environments let multiple users concurrently manage data, transform variables, perform exploratory analysis, build and compare models and score—with virtually no limits on the size of the data stored in Hadoop.
Big Data offers amazing opportunities for enterprises that take the right approach. With a well-thought-out Hadoop strategy organizations can gain a significant competitive edge.
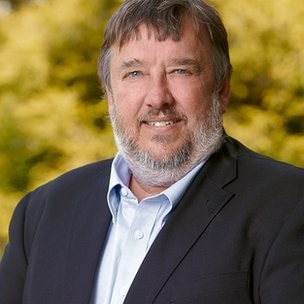
Paul Kent is the Vice President of Big Data at SAS. He coordinates with customers, partners and R&D teams to make sure the SAS development roadmap is taking world-class mathematics to the biggest baddest problems out there.
Paul joined SAS in 1984 as a Technical Marketing Representative and eventually moved into the Research and Development division. He has used SAS for more than 20 years and has contributed to the development of SAS software components including PROC SQL, the WHERE clause, TCP/IP connectivity, and portions of the Output Delivery System (ODS). A strong customer advocate, Paul is widely recognized within the SAS community for his active participation in local and international users conferences.
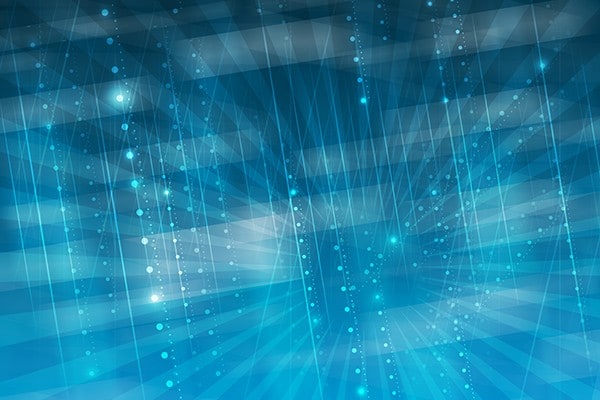
Read More
- What is Hadoop? Find out now.
- Read more Big Data Insights.
- Get the CIOReview magazine: Special Edition on Big Data (pdf).