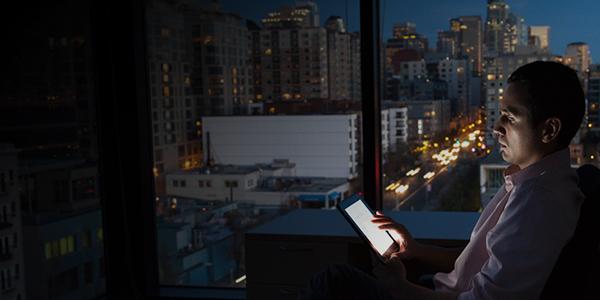
4 strategies that will change your approach to fraud detection
Fraudsters and money launderers are getting more sophisticated with their tactics. We’re getting more sophisticated at fighting back.
David Stewart, Business Director, Security Intelligence Solutions, Banking, SAS Security Intelligence Practice
Ian Holmes, Global Lead, Enterprise Fraud Solutions, SAS Security Intelligence Practice
Are financial institutions living in a riskier world or just growing more aware of the risks? It’s hard to say, but either way, the numbers are sobering. According to more than 7,000 respondents in PwC’s 2018 Global Economic Crime and Fraud Survey:
- Fraud is pervasive: 49 percent of global organizations report having been a victim of fraud and financial crime (up from 36 percent in 2016). And those are just the ones that know. The true figure is undoubtedly much higher.
- Fighting fraud is costly: 46 percent of respondents said their organizations spent the same or more on investigations and other interventions than was directly lost to the crime itself.
- Tuning fraud detection systems is a science that, when done wrong, wastes time and irritates customers: 34 percent of respondents said their financial crimes technologies produced too many false positives.
“The business case for investment in anti-fraud technology goes beyond protecting the organization from reputational, regulatory and/or financial damage,” said Didier Lavion, who headed up the study for PwC. “It also includes reducing the cost of fraud prevention through efficiencies and enabling an organization to safely build and sell new products and services on a digital platform.”
Fortunately, advances in fraud detection technologies are giving financial institutions a more accurate and efficient arsenal than ever for attacking fraud and financial crimes. Let’s take a look at four ways to turbocharge your defenses.
1. Bring new accuracy and efficiency to fraud detection with artificial intelligence.
Machine learning – a form of artificial intelligence (AI) – is a powerful force for improving both the accuracy and efficiency of fraud detection.
Find more fraud – earlier – with machine learning.
Unlike rules, which are easy for fraudsters to test and circumvent, the application of machine learning through analytics has been the standard for the SAS® fraud and financial crimes solutions for many decades.
- Supervised machine learning algorithms can self-learn from targets within the data, flag anything that doesn’t fit the observed norm, and then apply this knowledge to new and unseen data.
- Unsupervised machine learning uncovers potentially suspicious risks you might not think to look for, since it works without being given a target. Instead, it looks for anomalies in the data.
It’s easy to see how these techniques, combined in an ensemble model, provide a valuable breadth of coverage across current risks, as well as new and emerging threats.
Machine learning reduces false positives from existing approaches while identifying previously unknown risks. For example, SAS deployed a digital payment model that demonstrated rapid success for real-time fraud detection. It detected 50 percent of fraud, alerting on only 0.5 percent of the portfolio, with very few false positives.
Machine Learning Use Cases in Financial Crimes
Ten practical and achievable ways to put machine learning to work
Use machine learning to improve process efficiency.
Financial institutions want to reduce the costs of managing fraud and financial crimes operations that have risen to an unsustainable level given the speed and dynamic nature of attacks. With machine learning, systems can automatically:
- Create and update rules for detection and alert handling. Machine learning can examine masses of data to help establish rules and keep them current. Even something as simple as a decision tree can add some benefit (certainly in the segmentation approach) to more accurate rules. A typical branch of seven or eight nodes that points to a fraud-rich area in the data is a pretty easy discovery for a machine, but a very difficult one for a human.
- Select the most accurate detection models. For many years at SAS, we've used a combination of machine learning techniques to deliver the most accurate detection rates. Our approach allows newer techniques, like gradient boosting and support vector machines, to enhance proven methods such as neural networks. We now have interfaces that can intelligently launch 10,000 iterations of strategies so that the process is much more automated with limited human intervention.
- Automate processes for investigation. On average, 60 to 70 percent of an investigator’s time is spent collecting data about a subject. Machine learning can guide systems to automatically search and retrieve data, run database queries and collect information from third-party data providers without human intervention. We’re seeing clients reduce time to case decision by 20 to 30 percent.
Unlike rules, which are easy for fraudsters to test and circumvent, the application of machine learning through analytics has been the standard for the SAS® fraud and financial crimes solutions for many decades.Jim Goodnight CEO SAS
2. Converge fraud, anti-money laundering and cyber events.
Financial institutions are taking advantage of big data architectures to consolidate data across typically isolated functions. It only makes sense to bring these functions together for a more holistic view of risk. Much of the data is similar, regulations are pushing risk identification closer to real time, and there is abundant opportunity to reduce operational costs and enhance efficiency while developing a cross-functional view. In fact, regulators in some countries expect firms to have a holistic view of risk across functions and to report “cyber events” as part of their normal AML and fraud SAR reporting obligations.
Several SAS clients score transactions for multiple types of risk during a single engine process. Whether you’re reviewing a loan applicant, decisioning a payment transaction or uncovering terrorist financing, there are opportunities to learn from every interaction. Achieving real-time agility in a faster payments world is essential because retail and financial entities are adapting to meet customer demands for convenience, intensifying the risk environment:
- The rise of mobile and online activity has reshaped expectations for speedy authentication across all contact channels. You need a combination of customer, device and session behavioral analysis to prevent losses.
- More forward-thinking intelligence units are finding common elements – domain names, IP addresses, devices, etc. – that reveal transnational criminal organizations previously undiscovered by siloed functions.
- The demand for real-time fund availability began with the UK banking initiative Faster Payments Service (FPS), which reduces payment clearing times from three working days under the legacy BACS system to a few hours. New payments systems in the US and Australia – coupled with the growth of fintechs – are bringing this down to a few seconds.
Once a luxury, real-time transaction monitoring is now a baseline requirement for all payment types, incorporating not only financial transactions, but also authentication, session, location and device event data.
A tier one global bank reported that when it adopted SAS’ 100-percent real-time decisioning, it not only significantly reduced fraud, but also increased card revenue by $50 million in the first year of adoption due to lower false positive rates and improved customer experience.
3. Adopt more agile know your customer (KYC) processes.
High-profile leaks, such as the Panama Papers, dramatize the need for more transparency into the real owners or beneficiaries of corporate and legal entities. At the same time, the financial industry has seen deposit account and credit application fraud rise to 20 percent of all banking fraud. Both of these realities redefine the expectations for KYC processes. SAS is responding by:
- Fortifying and speeding authentication processes that validate digital devices and in-person applicants.
- Using robotic process automation (or RPA) to automate searches and queries of third-party data during an enhanced due diligence process.
- Supporting new data elements, such as ownership percentages and controlling interests.
- Offering investigative interfaces that streamline the ad hoc process of gathering external unstructured information, including numbers, text, images and video.
For document query and retrieval, we've been developing some interesting capabilities for image recognition using natural language processing that are showing remarkable results. In one test case, we reduced the time to identify, classify and analyze trade documents from 700 hours to a matter of minutes.
In another pilot, we scanned approximately 9,000 SWIFT messages looking for things such as Palestinian boycott language. A human would need about five to seven minutes to review each message. During this pilot we found we could do image recognition and contextual analysis of those messages in less than one second per message.
4. Streamline investigations with intelligent case management.
Much of the initial adoption of AI focuses on automating manual processes to reduce the costs of running a fraud and financial crimes front line. Investigators shouldn’t spend their valuable time on rote tasks that machines can do better. An advanced, analytics-driven alert and case management solution can automatically:
- Prioritize cases, recommend investigative steps and fast-track straightforward cases.
- Enrich alerts with detail about the associated customers, accounts or beneficiaries.
- Intelligently find and pull data for a case from internal databases or third-party data providers.
- Present data in easy-to-understand visualizations, appropriate for the type of activity under review.
- Allow automated prioritization of client contact strategies where suitable.
- Autopopulate and prepare SARs for electronic filing if applicable.
SAS has been automating operational processes for years, including alerts prioritization/triage, investigative processes and dashboards. Currently SAS is prototyping an adaptive learning system that embeds analytics into our alert and case management capabilities to automate processes and continuously learn from outcomes. This enables the system to adapt to new financial crimes risks. SAS continues to push the envelope on behavioral analytics, cognitive computing, and deep learning so that we have the most effective financial crimes risk platform available in the market.
About the Authors
David Stewart, Business Director, Security Intelligence Solutions, Banking, SAS Security Intelligence Practice
David Stewart manages strategy development, product management, and marketing counsel for SAS fraud and compliance solutions worldwide. In addition to working closely with many of the world’s leading financial institutions and regulatory agencies, he collaborates with SAS R&D and delivery teams to deploy industry best practices for financial crimes solutions.
Ian Holmes, Global Lead for Enterprise Fraud Solutions SAS Security Intelligence Practice
Ian Holmes provides fraud expertise to drive the enhancement, pre-sales and business implementation of SAS Fraud Management globally with current and potential customers. Before joining SAS in 2011, Holmes gained global fraud operations expertise during several years as Head of Card Fraud for the UK division of HSBC.
Recommended reading
-
Makale How AI and advanced analytics are impacting the financial services industryTop SAS experts weigh in on the topics that are keeping institutions up at night and fraudsters in a job.
-
Makale A guide to machine learning algorithms and their applicationsDo you know the difference between supervised and unsupervised learning? How about the difference between decision trees and forests? Or when to use a support vector algorithm? Get all the answers here.
-
Makale Medicaid and benefit fraud in 2018 and beyondTo curb the growing amount of Medicaid and benefit fraud and improper payments, agencies and their commercial counterparts need fraud and abuse detection systems with data management and analysis that can keep up and even stay one step ahead.
-
Makale Online fraud: Increased threats in a real-time worldOnline and mobile banking is convenient for customers -- and an opportunity for fraudsters. With fraud methods constantly evolving, an analytical approach is a must for banks seeking early, accurate detection.
Ready to subscribe to Insights now?