Making sense of streaming data in the Internet of Things
by Daniel Teachey, Insights Editor
The Internet of Things (IoT) touches many aspects of our lives. From wearables and connected appliances to cars, factories and retail stores, more and more devices constantly churn out data that gets collected. Somewhere.
The sheer number of “things” in the Internet of Things is impressive; moderate estimates suggest that more than 20 billion devices will be connected by 2020.
The biggest challenge with sensors occurs after signals have been detected. At that point, you must decide:
- Where do I collect the data being generated?
- What do I keep and what can be discarded?
- How can I use it?
Clearly, IoT isn’t merely a buzzword. The data from sensors in IoT devices is used by trillions of other devices, people, organizations and places. Opportunities abound for those that can quickly and effectively collect, process and analyze this massive amount of streaming data.
But step back before getting caught up in thinking about what you can do with IoT data. Before you do anything, you should decide what to do and when to do it.
Today’s device-driven world is forcing analytics to occur as fast as the data is generated – the essence of IoT analytics. In the white paper, Understanding Data Streams in IoT, we've explained how event stream processing (ESP) makes sense of data streaming from the IoT. The excerpt below outlines the origins of IoT, and where the data comes from that serves as the foundation for streaming analytics.
The early world of sensors
The first sensors appeared decades ago. These early sensors were designed to detect events or changes in quantities, then provide a corresponding output – generally as an electrical or optical signal. Soon appearing in everyday objects, like touch-sensitive elevator buttons and lamps that dim or brighten when you touch the base, such sensors weren’t necessarily connected to each other, or to the internet. Sensors like these have been used for many purposes over the years – in manufacturing, energy, robotics, cars, airplanes, aerospace and health care. To capture and collect the signals coming from sensors, the operational historian emerged. This database software application logs and stores historical, time-based data that flows from sensors. The data stores are optimized for time-dependent analysis, which happens after the data is stored – and they’re designed to answer questions like: “What was today’s standard deviation from hourly unit production?”
Historian technology often uses manufacturing standards and captures event data from hundreds of sensor types and other real-time systems. These dedicated data historians can survive harsh conditions, such as a production floor, so they continue capturing and storing data even if the main data store is unavailable. Historian software often includes complementary tools for reporting and monitoring on historic data, and can detect trends or correlations. When an issue is flagged, the system can alert an operator about the potential problem.
This used to be an advanced way to generate value out of sensor data. But with the rise of the IoT, the uses for sensors – and the data streaming from them – have become much more diverse.
The big data explosion and sensors in the Internet of Things
Since 2012, two major changes have shaken the sensor world – and caused the IoT market to mature more rapidly than before:
- Sensors shrank. Technological improvements created microscopic scale sensors, leading to the use of technologies like microelectromechanical systems (MEMS). This made sensors small enough to be embedded into unique places like clothing.
- Communications improved. Wireless connectivity and communication technologies have improved to the point that nearly every type of electronic equipment can provide wireless data connectivity. This has allowed sensors, embedded in connected devices, to send and receive data over a network.
Today, organizations are investing heavily to capture and store as much data as possible. But their bigger challenge is to extract valuable information from the data while it’s still in motion, as close as possible to the occurrence of the event. If you wait to analyze data after it’s stored, it takes too long to react. That could mean missing a new business opportunity or losing out to a competitor.
In many ways, the IoT promises to create a highly efficient world. But achieving it demands constant analysis of the state of events based on sensor and machine communications happening all around us. To take full advantage of data streams in the IoT, organizations must understand the exploding number of ways “big” IoT data needs to be filtered, mashed up, compared, contrasted, interpolated and extrapolated. Consider:
- Volume. Can you quickly access, integrate, store, process and analyze today’s massive amounts of data?
- Variety. New types of IoT data are still emerging. Can you manage all the different types of data and the varied formats – structured, unstructured, semistructured – on the fly?
- Velocity. Think about how quickly text, image and video data is generated by cellphone cameras, social media and devices like smart watches. That’s only a small part of the data tsunami. Can you act quickly enough to capture and analyze all that data?
- Veracity. In its raw form, IoT data is “dirty” – it hasn’t been filtered, validated, profiled or cleansed. Making IoT data trustworthy so it can be used as the basis for data-driven decision making calls for data management standards like data quality and data governance. Newer technologies, such as blockchain, can also be used to ensure the original data sources can be trusted.
To learn more about how event stream processing can make a difference for your IoT initiatives, download and read the white paper: Understanding Data Streams in IoT.
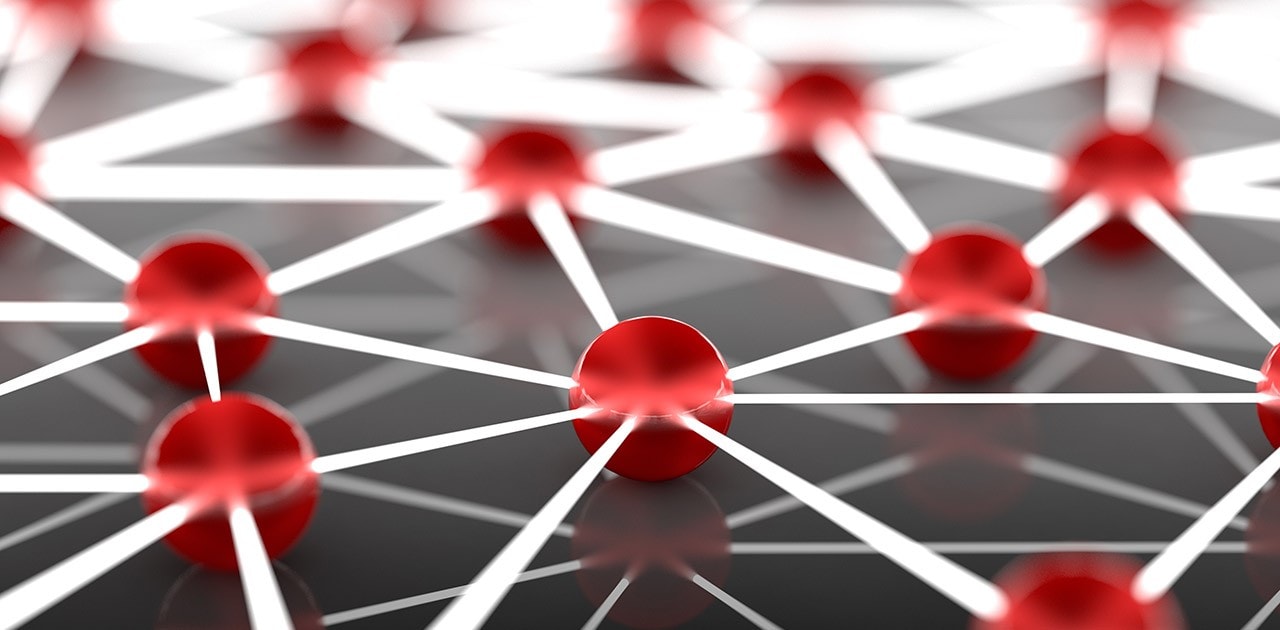
Get More Insights
Want more Insights from SAS? Subscribe to our Insights newsletter. Or check back often to get more insights on the topics you care about, including analytics, big data, data management, marketing, and risk & fraud.