Risk data infrastructure: Staying afloat on the regulatory flood
How to raise the bar and meet new reporting challenges
By Luis Jesus and David Rogers, SAS Risk Quantitative Solutions
Banks and financial institutions across the globe continue to face revised or new risk-related regulations as reporting requirements become more stringent. The European Banking Authority (EBA) COREP/FINREP regular taxonomy changes and the European Central Bank's (ECB's) recent requirements on environmental, social and governance (ESG) initiatives are just some of the drivers for ensuring granular storage with minimal latency and appropriate definitions for each data element.
Data volumes have increased significantly over the past few years. But the proliferation of data sources with granular specificity has resulted in tremendous data fragmentation across the enterprise. Today, data is more crucial than ever – yet even more difficult to access, manage and use.
Creating consistent and integrated data management processes has long been a vision for many financial institutions. The time to make this a reality is now. Indeed, the current regulatory environment challenges risk and financial silos to meet integrated reporting requirements – from simpler ad hoc reporting to more complex stress-testing exercises.
Why existing risk data infrastructures may be inadequate
Most banks embarked on creating a regulatory risk data infrastructure many years ago (for example, as part of their Basel II implementation). However, this risk data infrastructure was, in most cases, tactical and limited to meeting immediate requirements of the time. It’s often considered inadequate to accommodate the needs of newer regulations.
In January 2013, the Basel Committee on Banking Supervision (BCBS) published principles for risk data aggregation. These principles are structured around four broad areas: governance, data aggregation, risk reporting and supervisory requirements (Figure 1).
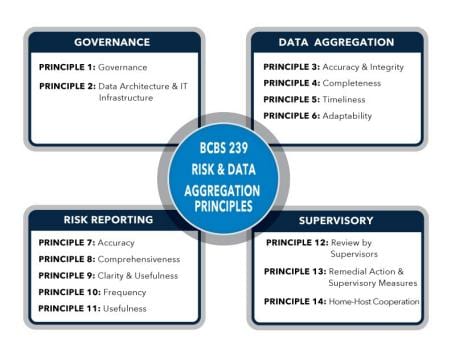
The BCBS 239 principles have become a reference across the banking industry and have elevated discussions about governance for risk data management from IT to senior management. However:
- The principles don’t provide operational guidelines for a bank to embark on a risk data infrastructure project.
- There are no accepted best practices or regulatory directives for appropriate risk data infrastructure that will help a bank meet various regulatory requirements from a data perspective.
Banks are still looking to improve their risk data infrastructure to reduce the costs of complying with existing regulations. At the same time, new regulations are adding to an already stressful situation by requiring even more granular data – not only for the current portfolio but also to support projections in stress testing exercises.
An ongoing process: Implementing risk data aggregation principles
As defined by BCBS, implementing the risk data aggregation principles should be a dynamic and ongoing process. This is due to the evolution of internal systems and processes as well as new data requirements – resulting, for example, from the evolution of the Basel framework and new reporting requirements around ESG. The Consultative Document: Principles for the effective management and supervision of climate-related financial risks – issued at the beginning of 2022 – requires that risk data aggregation capabilities and internal risk reporting practices account for climate-related financial risks.
According to a recent Basel Committee study, some progress has been made over the past few years. But considerable improvements are still needed. This is particularly the case for data architecture and IT infrastructure, which represent the building blocks for implementing the remaining risk and data aggregation principles.
The BCBS concludes that while there have been some improvements, only a small percentage of institutions in their analysis are fully compliant with the BCBS 239 principles. To address data quality challenges more comprehensively, some banks are changing their implementation approaches. (See Page 7 of this document by the Basel Committee on Banking Supervision to learn more.)
Multifaceted challenges
What are the challenges of a risk data infrastructure and how can banks address them? Consider the following examples.
- Multiple operational systems. Using disparate data warehouses or legacy IT systems generates poor quality data and makes aggregation difficult.
- Incomplete data. Banks often lack historical or detailed data. And it isn’t easy to access and combine data held in disparate systems.
- Data inconsistencies. Nonexistent, inconsistent, unintegrated or imprecise data dictionaries, data models, data taxonomies or definitions result in poor data quality that is time-consuming to fix.
- Lack of automation. Dependence on manually intensive processes or end-user computing without sufficient controls means there is no audit trail (or an incomplete trail). And testing of manual controls may be inadequate.
What if banks continue using disparate data warehouses or legacy IT systems along with unintegrated or imprecise data dictionaries, data models, data taxonomies or definitions? In this scenario, they will not be able to produce reports with the right level of accuracy and granularity.
How an enterprise risk and finance reporting warehouse can help
Banks can overcome the challenges of scattered, incomplete and inconsistent data by building an enterprise risk and finance reporting warehouse. Building a warehouse that incorporates data quality processes solves several issues and provides multiple advantages:
- Unified data. An enterprise risk data warehouse combines data from various operational systems and is an authoritative source for risk and finance reporting.
- Trustworthy data (i.e., data integrity). An enterprise data warehouse can be the single source of all data used for analytical applications and reporting. In turn, it will be much easier to reconcile results and reports to the warehouse and to source systems, such as those for accounting and trade capture. By reconciling and drilling into the data, banks can complete their supervisory reviews.
- Comprehensive data. The enterprise data warehouse supports data required for a variety of risk, regulatory and business issues – including Basel III, credit market, liquidity and ESG risks.
- Consistent data. Adding data governance processes on top of the risk data warehouse ensures that there are owners and stewards for all risk and finance data. This means that banks can track all data additions, changes or versioning. And a well-designed enterprise data warehouse would hold data history and offer data versioning.
- Reliable reporting and terminology. An enterprise data warehouse built with a comprehensive dictionary that describes banking data elements for all relevant balance-sheet and off-balance sheet items provides a complete mapping of physical data structures to business terms.
When it comes to risk, high-quality data is not an option. It is essential for implementing effective risk strategies and ensuring compliance.
Read more
Get More Insights
Want more Insights from SAS? Subscribe to our Insights newsletter. Or check back often to get more insights on the topics you care about, including analytics, big data, data management, marketing, and risk & fraud.