Analytics drives innovation
Three guideposts that lead to analytics success
by Fiona McNeill, Product Marketing, SAS
Being innovative is about the journey, not the destination. And in order to be innovative, companies need to guide themselves into an analytical path.
Why? By implementing analytically driven insight, monitoring analytically modeled outcomes and improving those models over time, an organization will continue to learn. Did that new customer target audience respond to the campaign? Was the increase in sales as forecasted? Was that transaction potentially a fraudulent act?
Converting the analytic environment into an operational process drives innovation. If the targeted customers didn’t respond as predicted – and based on their feedback and observed behavior since the campaign – you know that you need to communicate differently.
The organization’s strategy should direct the analytical environment, and the analytical environment should support the company’s strategy.
This relationship between organizational strategy and the analytical environment is built in three distinct stages. These stages may be performed at different points along an analytics maturity timeline, or they can occur simultaneously.
Stage 1: Long-term informational insight
This type of analysis is what helps organizations identify trends and evaluate business scenarios. Data warehousing, data marts, data lakes, virtualized repositories, online analytical processing (OLAP), reports and interactive visual analysis on loosely coupled sources typically support this stage.
At this stage, users are concerned with presenting information about past and current behavior (e.g., sales by region, branches, products) and, of course, changes that have occurred over time.
You can easily replace sales with any other business issue or element (churn, subscriptions, claims, investments, accounts, etc.). You can also replace other dimensions (region, branch and product) by any business factor you may be interested in analyzing. You can’t replace the dimension of time, however; time is always a constant in this exploratory analytical approach.
Often there is a production environment that provides source data for this kind of analytic effort, but while the data may be present, the ability to easily analyze it may not.
Analytics at this stage provides fact-based informational insights, helping to align the organization, orient the next action and even shape your strategy.
Stage 2: Internal and external environment mapping
This mapping can include market considerations, customers’ behaviors, competitors’ actions, and details regarding the products and services that your organization offers.
Questions explored in this stage include:
- How profitable are my products and services?
- How well have they been adopted by the target audience?
- How well do they suit my customers’ needs?
Statistical analysis helps provide the answers using correlations, topic identification and association statistics methods. And often there’s an analytical environment that’s made available to perform such queries.
This stage of analysis is performed on demand, when business departments request deep information about a particular business issue.
It differs from Stage 1 in that there typically isn’t a production environment to provide real-time answers. Nor is there a predefined web portal, or any other type of interface for rapidly responding to questions.
Stage 2 analytics provides likelihoods and degrees of accuracy associated with quantified patterns. This allows organizations to identify the merits of particular scenarios or characteristics that help direct efforts to improve or change current activity.
Stage 3: Corporate strategy alignment
The development and deployment of analytical models is directed by core business goals such as product expansion (with cross-sell or up-sell initiatives), attrition prevention, fraud identification and risk mitigation. Data- and text-mining models, forecasting and other methods that use artificial intelligence, machine learning or statistics commonly support these types of endeavors.
Supervised and unsupervised models are deployed to classify and predict particular events and to recognize groups of similar behaviors. Success of the effort is monitored to evaluate if the subscribed business change occurred, and if not, adjustments are made.
Stage 3 analytics provides scenario detection for guiding future operational and organizational actions.
At its core, analytics provide a foundation for data-driven innovation, creating and delivering new knowledge, and accessible information. Each of these stages might support insights and decisions for different members of the organization, based on their roles and responsibilities.
The difference with analytically mature, innovative organizations, however, is that these insights are universally accessible – whether you work in HR, finance, sales, logistics, marketing or services. The data is recognized as a corporate asset and analytical methods become intellectual property.
Innovation demands change
Innovation means we make a conscious effort to address a particular situation and put into place something that may never have been tried before. It means pursuing an idea and determining if it is valid, feasible, consistent, understandable, comprehensive and applicable.
Innovation is a trial-and-error process. And in this respect, innovation means to try – sometimes getting it right and making things better, and sometimes not.
Fiona McNeill is Global Product Marketing Manager at SAS and oversees the product marketing and messaging for specific SAS technologies. With a background in applying analytics to real-world business scenarios, she focuses on the automation of analytic insight in both business and application processing. She has worked with organizations across a variety of industries, understanding their business and helping them derive tangible benefit from their strategic use of technology.
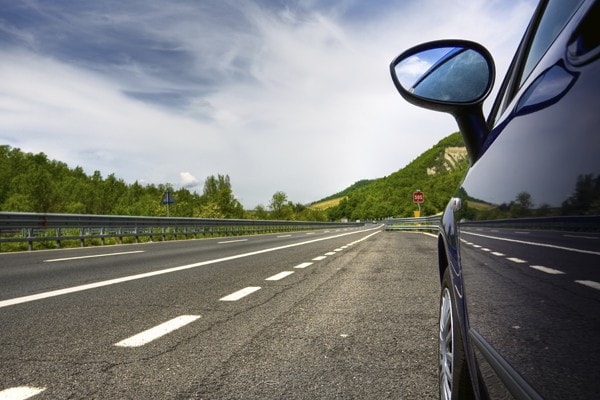
Read More
- The new book by Fiona McNeill and co-author, Carlos Pinheiro, The Heuristics in Analytics Is now available.
- Return to Analytics Insights
Book Excerpt
- Read the first chapter of Heuristics in Analytics: A Practical Perspective of What Influences Our Analytical World.