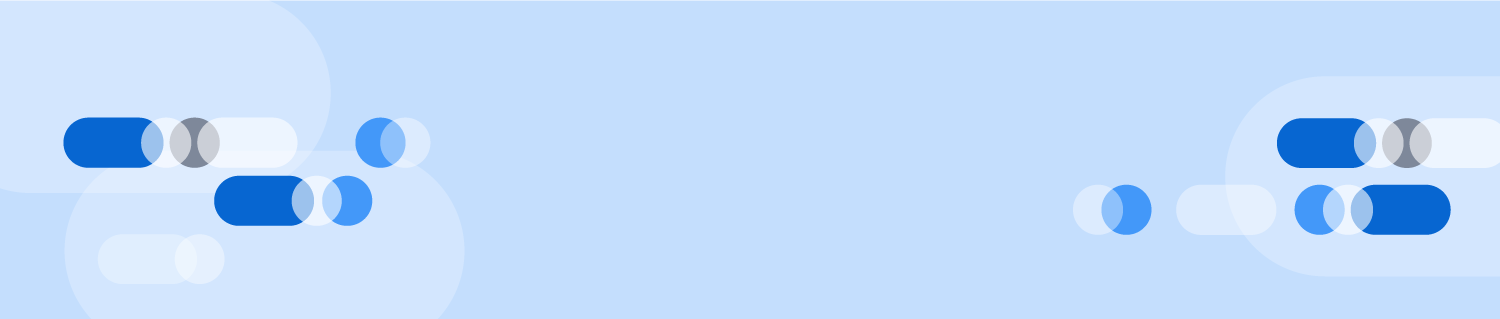
Data Mining
Was ist das und was man darüber wissen sollte
Bei Data Mining handelt es sich um einen Prozess, mit dessen Hilfe man Besonderheiten, Strukturen und Wechselbeziehungen innerhalb großer Datensätze erkennen kann und somit in der Lage ist, Zukunftsprognosen zu stellen. Durch Nutzung eines breiten Technologiespektrums können Sie diese Informationen zur Umsatzsteigerung, Kostensenkung, Verbesserung Ihrer Kundenbeziehungen, Risikominimierung und für viele weitere Maßnahmen nutzen.
Data Mining Geschichte & aktuelle Fortschritte
Das Durchsuchen von Daten, um verborgene Zusammenhänge zu entdecken und zukünftige Trends vorherzusagen, hat eine lange Geschichte. Der Begriff "Data Mining", der manchmal auch als " Erkennung von Wissen in Datenbanken" bezeichnet wird, wurde erst in den 1990er Jahren geprägt. Seine Grundlage bilden jedoch drei miteinander verwobene wissenschaftliche Disziplinen: Statistik (die numerische Untersuchung von Datenbeziehungen), künstliche Intelligenz (menschenähnliche Intelligenz, die von Software und/oder Maschinen dargestellt wird) und maschinelles Lernen (Algorithmen, die aus Daten lernen und Vorhersagen treffen können). Was alt war, ist wieder neu, denn die Data-Mining-Technologie entwickelt sich ständig weiter, um mit dem grenzenlosen Potenzial von Big Data und erschwinglicher Rechenleistung Schritt zu halten.
In den letzten zehn Jahren haben wir dank der Fortschritte im Bereich Prozessorleistung -und Geschwindigkeit die Möglichkeit, manuelle, sehr mühsame und zeitaufwändige Verfahren durch schnelle, einfache und automatische Datenanalysen abzulösen. Je komplexer die gesammelten Datensätze sind, desto größer ist die Wahrscheinlichkeit, aussagekräftige und fundierte Schlüsse ziehen zu können.
Der Einzelhandel, Banken, Fertigungsindustrie, Telekommunikationsunternehmen und Versicherungen nutzen Data Mining, um Zusammenhänge zu erkennen, die von der Preisoptimierung über Werbeaktionen und demografische Daten bis hin zu den Auswirkungen von Wirtschaft, Risiken, Wettbewerb und sozialen Medien auf ihre Geschäftsmodelle, Einnahmen, Abläufe und Kundenbeziehungen reichen.
Why is data mining important?
So why is data mining important? You’ve seen the staggering numbers – the volume of data produced is doubling every two years. Unstructured data alone makes up 90 percent of the digital universe. But more information does not necessarily mean more knowledge.
Data mining allows you to:
- Sift through all the chaotic and repetitive noise in your data.
- Understand what is relevant and then make good use of that information to assess likely outcomes.
- Accelerate the pace of making informed decisions.
- Uncover new insights from data through the use of predictive analytics.
Warum ist Data Mining wichtig?
Warum ist Data Mining also wichtig? Sie haben die schwindelerregenden Zahlen gesehen - die Menge der produzierten Daten verdoppelt sich alle zwei Jahre. Allein die unstrukturierten Daten machen 90 Prozent des digitalen Universums aus. Aber mehr Informationen bedeuten nicht unbedingt mehr Wissen.
Data Mining ermöglicht Ihnen:
- Das chaotische und sich wiederholende Rauschen in Ihren Daten zu durchforsten
- Verstehen, was relevant ist, und diese Informationen dann sinnvoll nutzen, um voraussichtliche Ergebnisse zu bewerten
- Den Entscheidungsprozess zu beschleunigen, um fundierte Geschäftsentscheidungen zu treffen
Data Mining in Today's World
Data mining is a cornerstone of analytics, helping you develop the models that can uncover connections within millions or billions of records. Learn how data mining is shaping the world we live in.
Data Mining-Software
Data Mining-Software von SAS nutzt bewährte, hochmoderne Algorithmen, mit denen Sie selbst die größten Herausforderungen bewältigen können.
Wer nutzt Data Mining?
Für viele Branchen und Fachbereiche ist Data Mining das Herzstück der Analyseverfahren.
Telekommunikation, Medien und Technologie
In einem vom Wettbewerb geprägten, unüberschaubaren Markt liegen die Antworten oft in Ihren Kundendaten. Telekommunikations-, Medien- und Technologieunternehmen können mit Hilfe von Analysemodellen Unmengen von Kundendaten auswerten, um das Kundenverhalten vorherzusagen und zielgerichtete und passende Angebote anzubieten.
Versicherungswesen
Mit analytischem Know-how können Versicherungsunternehmen komplexe Probleme in Bezug auf Betrug, Einhaltung von Vorschriften, Risikomanagement und Kundenabwanderung lösen. Unternehmen nutzen Data-Mining-Techniken, um die Preise für Produkte in verschiedenen Geschäftsbereichen zu optimieren und neue Wege zu finden, ihrem bestehenden Kundenstamm wettbewerbsfähige Produkte anzubieten.
Bildung
Mit einer einheitlichen, datengestützten Übersicht über die Fortschritte der Schüler können Pädagogen die Leistungen ihrer Schüler, bevor diese überhaupt das Klassenzimmer betreten haben, vorhersagen und somit bereits Interventionsstrategien entwickeln, um die Schüler auf Kurs zu halten.
Mit Hilfe von Data Mining können Pädagogen auf Schülerdaten zugreifen, Leistungsniveaus vorhersagen und Schüler oder Schülergruppen identifizieren, die besondere Aufmerksamkeit benötigen.
Fertigungsindustrie
Die Abstimmung der Beschaffungspläne mit den Bedarfsprognosen ist ebenso wichtig wie frühzeitige Erkennung von Problemen, die Qualitätssicherung und Investitionen in den Markenwert. Hersteller können die Verschleißerscheinungen von Fertigungsanlagen vorhersagen und Wartungsarbeiten berücksichtigen, wodurch die Maschinenlaufzeit maximiert und die Produktionslinie im Zeitplan gehalten werden kann.
Bankwesen
Automatisierte Algorithmen helfen Banken, ihren Kundenstamm sowie die Milliarden von Geschäftsvorgängen im Herzen des Finanzsystems zu verstehen. Data Mining hilft Finanzdienstleistern, einen besseren Überblick über Marktrisiken zu erhalten, Betrugsfälle schneller zu erkennen, die Einhaltung gesetzlicher Vorschriften zu verwalten und eine optimale Rendite für ihre Marketinginvestitionen zu erzielen.
Einzelhandel
Große Kundendatenbanken enthalten wertvolle Informationen über Kunden, die Ihnen helfen können, Beziehungen zu verbessern, Marketingkampagnen zu optimieren und Umsatzprognosen zu erstellen. Durch genauere Datenmodelle können Einzelhandelsunternehmen gezieltere Aktionen anbieten - und das Angebot finden, das die größte Wirkung auf den Kunden hat.
Erfahren Sie mehr über Branchen, die diese Technologie nutzen
Wie funktioniert Data Mining
Als eine Mischung unterschiedlicher Technologien, steht Data Mining für eine Vielzahl von Methoden oder Techniken, die in verschiedenen Analysemöglichkeiten zum Einsatz kommen, die eine Reihe von organisatorischen Anforderungen abdecken, verschiedene Arten von Fragen stellen und in unterschiedlichem Maße menschliche Eingaben oder Regeln verwenden, um eine Entscheidungsgrundlage zu schaffen.
Descriptive Modeling: Dieses Verfahren deckt Gemeinsamkeiten oder Zusammenhänge in der Datensammlung auf, um Ursachen für Erfolg bzw. Misserfolg zu ermitteln, z. B. eine Zuordnung nach Produktvorlieben- oder Zufriedenheit bei Kunden. Folgende Methoden sind als Beispiele zu nennen:
Clustering | Gruppierung ähnlicher Datensätze |
Anomalie-Erkennung
| Identifizierung mehrdimensionaler Ausreißer
|
Erlernen von Assoziationsregeln
| Erkennen von Beziehungen zwischen Datensätzen
|
Analyse der Hauptkomponenten
| Erkennen von Beziehungen zwischen Variablen
|
Eingruppierung nach Affinitäten | Eingruppierung von Personen mit gemeinsamen Interessen oder ähnlichen Zielen (z. B. Personen, die X kaufen, kaufen häufig Y und möglicherweise Z) |
Predictive Modelling: Diese Modellierungsverfahren geht stärker in die Tiefe. Es dient der Klassifikation künftiger Ereignisse oder der Einschätzung unbekannter Ergebnisse. Ein Beispiel dafür ist das Credit Scoring, mit dem die Wahrscheinlichkeit ermittelt wird, dass eine Person einen Kredit ordnungsgemäß abbezahlt. Predictive Modelling hilft auch bei der Erkennung von Faktoren wie Kundenfluktuation, Reaktion auf Kampagnen oder Kreditausfälle. Verfahren sind beispielsweise:
Regressionverfahren | Ein Messverfahren für die Beziehungsstärke zwischen einer abhängigen Variablen und einer Reihe unabhängiger Variablen |
Neurale Netzwerke | Lernfähige Computerprogramme für Mustererkennung und Prognosen |
Entscheidungsbäume | Diagramme mit einer Baumstruktur, bei der jeder Zweig für ein mögliches Ereignis steht |
Support Vector Machines (SVMs) | Überwachte Lernmodelle mit zugehörigen Lernalgorithmen |
Prescriptive Modelling : Die wachsenden Mengen unstrukturierter Daten aus dem Internet, aus Kommentarfeldern, Büchern, E-Mails, PDF-Dateien, Audio- und anderen Textquellen verhelfen dem Text Mining als verwandter Disziplin des Data Mining zu steigender Bedeutung. Denn man muss in der Lage sein, unstrukturierte Daten erfolgreich zu analysieren, zu filtern und zu transformieren, um sie in Vorhersagemodellen für genauere Prognosen nutzen zu können.
Letztendlich sollte man Data Mining nicht als separate, eigenständige Technik betrachten, da Vorbereitung (Datenvorbereitung, Data Exploration) und Nachbereitung (Modellprüfung, Scoring, Modellleistungsüberwachung) gleichermaßen unverzichtbar sind. Prescriptive Modelling generiert auf der Basis interner und externer Variablen und Beschränkungen eine oder mehrere Handlungsempfehlungen – beispielsweise das jeweils beste individuelle Marketing-Angebot für jeden Kunden. Verfahren sind beispielsweise:
Predictive Analytics plus Regeln | Entwicklung von Wenn-dann-Regeln auf der Grundlage von Mustern sowie Vorhersage der Ergebnisse |
Marketing Optimization | Simulation des vorteilhaftesten Medienmix in Echtzeit für den höchstmöglichen ROI |
Weitere Informationen zu diesem Thema
-
What is AI modeling?AI modeling involves creating programs that use one or a combination of algorithms to allow computers to think, learn and predict outcomes. Much like a human brain, AI models absorb input data – numbers, texts, images, video, sound – to learn how to predict outcomes or solve specific tasks without explicit instructions at every step.
-
4 strategies that will change your approach to fraud detectionAs fraudulent activity grows and fighting fraud becomes more costly, financial institutions are turning to anti-fraud technology to build better arsenals for fraud detection. Discover four ways to improve your organization's risk posture.
-
Machine learning and artificial intelligence in a brave new worldWhat is the interplay between man and machine in a brave new world with AI?
-
Your personal data scientistImagine pushing a button on your desk and asking for the latest sales forecasts the same way you might ask Siri for the weather forecast. Find out what else is possible with a combination of natural language processing and machine learning.