Big data and global development
A primer on using online and mobile data to make the world a better place
The data revolution is not restricted to the industrialized world. The spread of mobile phone technology into the hands of billions of individuals may be the single most significant innovation that has affected developing countries in the past decade.
Across the developing world, mobile phones are used daily to transfer money, buy and sell goods, and communicate information including test results, stock levels and prices of commodities. Mobile technology is used as a substitute for weak telecommunications and transport infrastructures as well as underdeveloped financial and banking systems.
The numbers of real-time information streams and people using social media are growing rapidly in developing countries as well. Tracking trends in online news or social media can provide insights on emerging concerns that can be highly relevant to global development.
The recent waves of global shocks – food, fuel and financial – have led to greater volatility, and policymakers are increasingly aware of the social and financial impact. Despite greater interconnectivity, local impacts of shocks like food crises or natural disasters may not be immediately visible and trackable.
These are important issues that often unfold beneath the radar of traditional monitoring systems, and by the time hard evidence finds its way to the front pages of newspapers and desks of decision makers, it’s often too late and more expensive to respond. While early-warning systems and data collected through “traditional” methods (surveys and statistics) continue to generate relevant information, the digital revolution presents a tremendous opportunity to gain richer insight into the human experience, and big data can complement the existing indicators.
What is big data for development?
“Big data for development” is a concept that refers to the identification of sources of big data relevant to the policies and planning for development programs. It differs from both “traditional” development data and what the private sector and mainstream media call “big data.”
In general, sources of big data for development are those which can be analyzed to gain insight into human well-being and development, and generally share some or all of the following features:
Sources of big data for development are those which can be analyzed to gain insight into human well-being and development
- Digitally generated: Data is created digitally, not digitized manually, and can be manipulated by computers.
- Passively produced: Data is a by-product of interactions with digital services.
- Automatically collected: A system is in place that automatically extracts and stores the relevant data that is generated.
- Geographically or temporarily trackable: For instance, this is the case in mobile phone location data or call duration time.
- Continuously analyzed: Information is relevant to human well-being and development, and can be analyzed in real time.
Big data for development is constantly evolving. However, a preliminary categorization of sources may reflect:
- What people say (online content): International and local online news sources, publicly accessible blogs, forum posts, comments and public social media content, online advertising, e-commerce sites and websites created by local retailers that list prices and inventory.
- What people do (data exhaust): Passively collected transactional data from the use of digital services such as financial services (including purchase, money transfers, savings and loan repayments), communications services (such as anonymized records of mobile phone usage patterns) or information services (such as anonymized records of search queries).
Before it can be used effectively, big data needs to be managed and filtered through data analytics - tools and methodologies that can transform massive quantities of raw data into “data about the data” for analytical purposes. Only then it is possible to detect changes in how communities access services that may be useful proxy indicators of human well-being.
If properly mined and analyzed, big data can improve the understanding of human behavior and offer policymaking support for global development in three main ways:
- Early warning: Early detection of anomalies can enable faster responses to population in times of crisis.
- Real-time awareness: Fine-grained representation of reality through big data can inform the design and targeting of programs and policies.
- Real-time feedback: Adjustments can be made possible by real-time monitoring of the impact of policies and programs.
Global Pulse is a United Nations innovation initiative of the Secretary-General, exploring how big data can help policymakers gain a better understanding of changes in human well-being. Through strategic public-private partnerships and R&D carried out across its network of Pulse Labs in New York, Jakarta and Kampala, Global Pulse functions as a hub for applying innovations in data science and analytics to global development and humanitarian challenges.
Big data analytics is not a panacea for age-old development challenges, and real-time information does not replace the quantitative statistical evidence that governments traditionally use for decision making. However, it does have the potential to inform whether further targeted investigation is necessary, or prompt immediate response.
Big data and global development examples
To evaluate the effectiveness of harnessing big data for development, UN Global Pulse has worked on several research projects in collaboration with public and private partners. Such proof-of-concept projects and prototypes demonstrate how big data analysis can be beneficial to the work of policymakers in different contexts – from monitoring early indicators of unemployment hikes to tracking fluctuations of commodity prices before they are recorded in official statistics. Detailed reports for these projects are also available on the Global Pulse site.
Social media as early indicator of an unemployment spike
Challenge: Can social media add depth to unemployment statistics?
Solution: Collect digital data (social media, blogs, forums and news articles) related to unemployment. Perform sentiment analysis to categorize the mood of these online conversations. Correlate volume of mood-related conversation to official unemployment statistics.
Results: Global Pulse and SAS found that increased social media conversations about work-related anxiety and confusion provided a three-month early warning indicator of an unemployment spike in Ireland.
Monitoring the evolution of food security issues through news media
Challenge: Is it possible to track thematic shifts in media attention through the automatic analysis of news articles?
Solution: Collect a corpus of news media on topics of interest, based on keywords (i.e., food security). Cluster articles in theme-based categories through semantic analysis. Visualize the information both geographically and over time.
Results: Thanks to the machine classification of millions of documents without human intervention, it’s possible to visualize the shift in media attention related to a specific topic (in this case, food security) over time and thematically.
Real-time tracking of commodity prices: the e-bread index
Challenge: Can mining online food prices provide real-time information on commodity price dynamics?
Solution: Use Web-scraping technologies to create a real-time price index ("e-bread index") by extracting bread prices from online supermarkets and retail websites. Compare this e-bread index with the official food price index.
Results: The relationship between Web-extracted prices and official statistics on food prices proved to be closely correlated, allowing for price forecasting and additional real-time indicators of inflation activity.
Twitter and perceptions of crisis-related stress
Challenge: Can Twitter data provide insight about issues related to food, fuel, housing and the economy in Indonesia?
Solution: Develop a taxonomy of keywords related to food, fuel, housing and the economy, as well as keywords reflective of concern. Classify Twitter messages into categories and quantify sentiment of relevant messages. Correlate or compare the volume of keywords from Twitter against official statistics and significant events.
Results: The number of tweets discussing the price of rice in Indonesia closely matched the official inflation statistics, showing how the volume and topics of Twitter conversations can reflect a population’s concerns in close to real time.
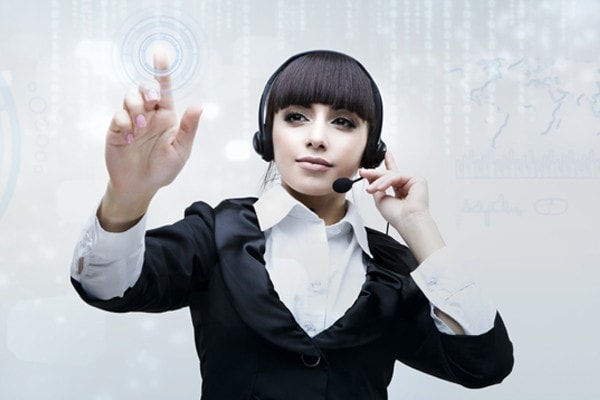
Read More
- Get more big data insights.
- Discover more about data for good.