Your personal data scientist
Introducing an analytical assistant for the masses
By Alison Bolen, SAS Insights Editor
My kids love Siri, Apple’s virtual assistant. They ask her about homework, sports scores and science experiments. They ask her questions that range from the mundane (What is your favorite kind of dog?) to the profound (What is free will?). And, within reason, she answers.
I’m a more practical user of Siri. I ask her to look up recipes, type texts, check my calendar and find directions all while I’m busy with other activities, like driving or cooking or chasing my kids.
Siri and similar systems – like the Amazon Echo, Google Now and Microsoft’s Cortana – have been described as personal assistants, and they’re becoming quite commonplace in our everyday lives. If you watch the popular video introducing the Amazon Echo, you see a family using that service throughout the day to keep grocery lists, set reminders and play music.
Powered partly by natural language processing and machine learning, these systems translate your spoken words into a computational query, find an answer among billions of data points, and provide the answer back in the same language you used to ask the question.
What if Siri were a data scientist?
The next step in the evolution of these personal assistants, especially for business decision makers, might be to use them to query large corporate data sets or even public data sets like social media feeds and government data.
Imagine pushing a button on your desk or your smartphone and asking any of the following questions:
- What are the sales forecasts through the end of this year for our 10 largest regions?
- What are the top three adjectives that customers are using to describe our new product on social media today?
- Which marketing programs are generating the most income this quarter?
- Show me the problems that customers are contacting us about this week through all of our customer contact channels.
The goal for these systems would be to put analytics into the hands of the masses. Data scientists are still needed to build models and to develop complex algorithms, but this type of system makes analytics more accessible to the average person.
Wayne Thompson
Chief Data Scientist
SAS
Using natural language processing, your personal data scientist turns your request into a query, searches all known databases for an answer, applies analytics to the data, and presents you with an answer – and perhaps a chart or data visualization that you can then drill into further.
“What we’re describing is a cognitive learning system that uses automation and natural language process questions and answers,” says Wayne Thompson, Chief Data Scientist at SAS. “Machine learning helps in the process of articulating how to return results and interpreting what other things are not explicitly requested that would make sense for the purpose of representing results to the user.”
What else would you like to know?
After you’ve evaluated the initial results, your personal data scientist can provide links to related answers or charts to encourage further exploration. The system might say, “Would you also like to know what customers are saying about competitors on social media?” Or, “Would you like me to forecast sales into next year?”
These prompts help can help you explore the data further, especially if you are not familiar with the data or the available types of analyses.
Sharing your results
And finally, if your query is new or related to something that somebody else has asked, your personal data scientist might say, “Would you like me to share these results to the portal?” Or, “Would you like to share these results with the VP of sales?” That way, important information is spread further into the organization so that others with an interest can benefit from it as well.
“Data scientists have the acumen and the ability to process data and know what to look for in specific types of data sets,” says Mike Frost, a Senior Product Manager at SAS. The goal of a personal data scientist would not be to replace these specialized people but to bring some of their more basic skills to the masses.
Thompson, a data scientist himself, is not afraid of being replaced. “The goal for these systems would be to put analytics into the hands of the masses. Data scientists are still needed to build models and to develop complex algorithms, but this type of system makes analytics more accessible to the average person.”
Next steps from your personal data scientist
If you don’t know what to ask of your data, you could also input a general topic by saying, “I’m interested in our supply chain,” and the system would share some basic insights on that topic and provide tips for further investigation.
No matter how you pose the question, natural language processing takes your statement and turns it into a series of queries the computer can understand. Machine learning processes the request, returns the results and looks for related things that are not explicitly requested, but might make sense to the requestor. Essentially, the system is predicting what other things you might ask next.
For some complex questions, your personal data scientist might produce a gallery of charts or results, to give you multiple views into the data and provide tips to probe deeper into the data.
The personal data scientist is a low level BI data discovery tool that learns the more you use it. It uses prebuilt machine learning models and maps the request from the user into a pipeline of models and data. The more you interact with the system to fine-tune your requests, the more it continues to learn.
What would you ask of a personal data scientist? And how can you see it being used to spread the use of analytics in your industry?
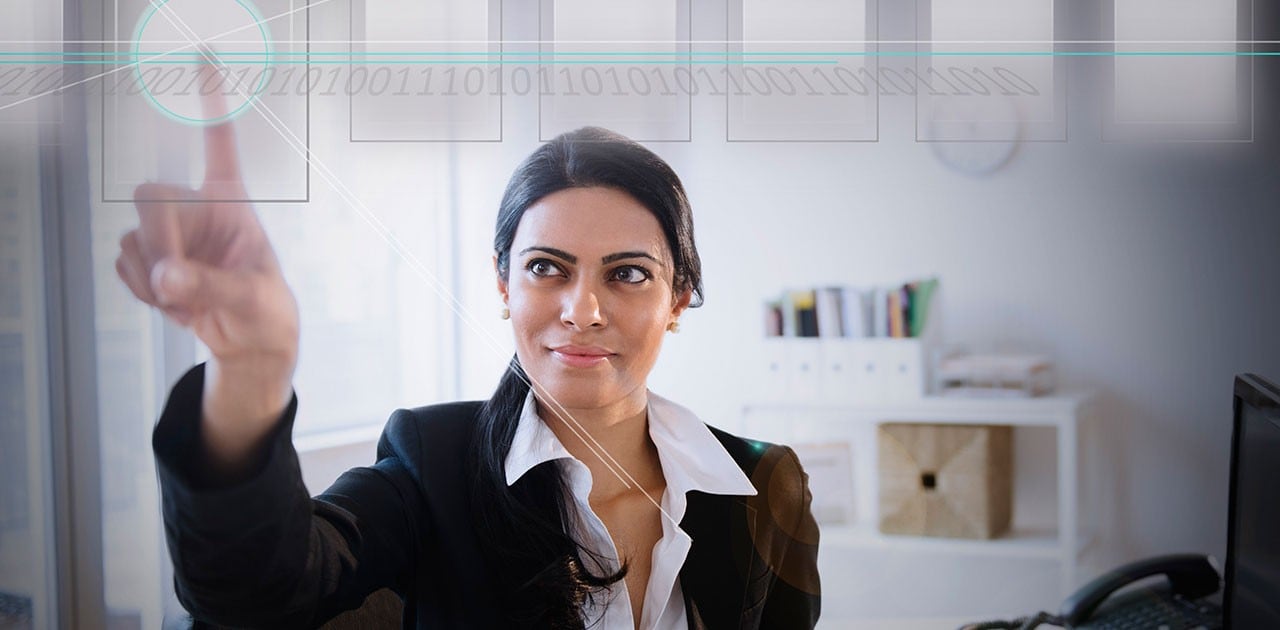
What To Read Next
- What is machine learning? And how is it used today? Find out in our machine learning overview.
- Learn more about natural language processing on the SAS blogs.
Get More Insights
Want more Insights from SAS? Subscribe to our Insights newsletter. Or check back often to get more insights on the topics you care about, including analytics, big data, data management, marketing, and risk & fraud.